Or try one of the following: 詹姆斯.com, adult swim, Afterdawn, Ajaxian, Andy Budd, Ask a Ninja, AtomEnabled.org, BBC News, BBC Arabic, BBC China, BBC Russia, Brent Simmons, Channel Frederator, CNN, Digg, Diggnation, Flickr, Google News, Google Video, Harvard Law, Hebrew Language, InfoWorld, iTunes, Japanese Language, Korean Language, mir.aculo.us, Movie Trailers, Newspond, Nick Bradbury, OK/Cancel, OS News, Phil Ringnalda, Photoshop Videocast, reddit, Romanian Language, Russian Language, Ryan Parman, Traditional Chinese Language, Technorati, Tim Bray, TUAW, TVgasm, UNEASYsilence, Web 2.0 Show, Windows Vista Blog, XKCD, Yahoo! News, You Tube, Zeldman
Parasoft C/C++test adds AI assistant | InfoWorld
Technology insight for the enterpriseParasoft C/C++test adds AI assistant 18 Jun 2025, 7:02 pm
Parasoft has released Parasoft C/C++test 2025.1, an update to the company’s automated testing solution for for C and C++ developers that introduces an AI assistant. The new release also adds nearly 70 static analysis rules, among other improvements.
The new AI assistant in Parasoft C/C++test 2025.1 facilitates rapid access to crucial information in Parasoft product documentation and user manuals based on task-focused inquiries, Parasoft said. The AI assistant is intended to increase overall C/C++ test usability and developer productivity while decreasing time and effort training new users on static and dynamic testing. The AI assistant complements the Parasoft GenAI agent, which integrates with the Visual Studio Code to fix static analysis violations, according to Parasoft. Users can configure the application to use a large language model provider of their choice. OpenAI, Azure OpenAI, and other LLM providers with similar functionality are supported, Parasoft said.
Parasoft C/C++test 2025.1 expands its static application security testing (SAST) capabilities by adding nearly 70 static analysis rules targeting Common Weakness Enumerations (CWEs), Parasoft said. The update boosts compliance with security standards such as ISO 21434 and IEC 62304 by identifying critical vulnerabilities such as memory corruption and logic flaws early in development, when these are less costly to fix.
A new test configuration option allows development teams to automatically suppress violations of equivalent rules, making it easier to work with multiple coding standards or to transition from one coding standard to another, Parasoft said. Previously, the same coding issue might be reported under a different rule ID than in the new standard, forcing developers to manually suppress violations that already had been suppressed.
Parasoft C/C++test 2025.1 also adds complete support for the MISRA (Motor Industry Software Reliability Association) C:2025 coding guidelines, and updates the rules for MISRA C:2023, removing obsolete rules and offering functional safety improvements. MISRA is a collaboration between manufacturers, component suppliers, engineering consultancies, and academics seeking to promote best practice in developing safety- and security-related electronic systems and other software-intensive applications, according to Parasoft.
Parasoft also the expanded compiler support to include new versions of ARM Compiler, Clang C/C++ Compiler, GNU GCC, Hexagon Clang Compiler, and Intel Classic C/C++ Compiler.
Retail versus finance: How genAI coding strategies diverge 18 Jun 2025, 4:20 pm
An AI security vendor on Tuesday published an analysis of the generative AI (genAI) coding differences between its retail and finance customers, revealing that the retail approach is much more aggressive, whereas finance has been working on the technology longer.
“Retail is pushing genAI into production faster. Finance is still experimenting. Retail companies are embedding genAI at 2.1 times the rate of financial services, based on the proportion of repositories that include genAI components,” said the report from AI vendor Apiiro, whose clients include Colgate-Palmolive, Shell Global, BlackRock, and the Rakuten Group.
The analysis, based on an examination of more than 100,000 code repositories using Apiiro’s Deep Code Analysis tool, showed how differently the two verticals are approaching genAI coding strategies. It noted, “61% of retail genAI repositories show active development, based on commit activity and contributor engagement. In financial services, that number [is] 22%. Retail teams are moving genAI projects through the build-test-ship cycle, while many finance teams remain in slower, more siloed experimentation phases.”
Much of the difference, the report said, comes from the tasks each vertical is pushing. “Retail teams are using genAI to power real-time, customer-facing features like recommendation engines and automated support. With shorter feedback loops and direct revenue impact, the incentive to ship is constant. Financial institutions, by contrast, operate under heavier regulatory scrutiny. Their genAI work is more cautious, often confined to internal systems and it shows in the development patterns.”
Financial services organizations, it said, have been working on genAI projects much longer, which is reflected in the ages of their repositories; the average age of a finance organization’s genAI repository is 688 days, significantly older than retail’s 453-day average.
Jason Andersen, VP and principal analyst at Moor Insights & Strategy, mostly agreed with the report’s findings and said the details matched his observations with clients in those two verticals.
He was intrigued by the age difference between the two sectors’ genAI repositories, but only because he would have guessed that retail would have been more of a laggard.
With finance’s “688 days, that’s roughly two years, which is just when most of the early (genAI) models started coming out,” Andersen said. “That makes sense because finance historically trends faster. They [understand] data better. I am amazed that it was 453 days for retail. I thought it would be much less. I think retail is moving even slower than that.”
The report said that retail genAI use cases typically involve customer-facing personalized product recommendations, automated support, and tailored promotions.
“These systems rely on real-time, user-specific context — and that means direct access to sensitive data,” it said. “In finance, genAI usage remains more siloed, less client-facing: pilot programs, internal assistants or data-abstracted training scenarios. Regulatory pressure plays a role, but so does engineering culture: [finance] pipelines are less often wired directly into live user data.”
Moor’s Andersen said that the industry priorities of the financial vertical means that financial IT “can be a lot more experimental” than its retail counterparts.
Financial “has more means, more money. That entire [financial] industry is based on beta so they are well primed for [genAI experimentation].”
Retail IT attacks these developments the same way that it always has. “They look at every automation move the same since the tractor,” Andersen said. “Retail IT asks, ‘How am I going to use this to increase margins?’ And financial is looking at [genAI] for innovation. They are asking, ‘How do I create new products?’”
Another area the report explored was tool usage.
“Financial services teams use a wide range of genAI tools, including OpenAI Client, LangChain, and LiteLLM. Their projects span multiple model types and dataset formats, which is a sign of active experimentation across varied use cases,” the report said. “Retail, by contrast, has converged on a smaller, tighter stack: OpenAI Python SDKs and LiteLLM dominate. These tools feed into high-leverage, customer-facing use cases like product recommendations and personalized search.”
Retail’s use of fewer tools, the report said, has the benefit of sharply accelerating operationalization.
“Fewer tools mean fewer integration points, tighter pipelines, and more repeatable patterns, [whereas] finance’s broader stack creates fragmented risk surfaces and steeper governance complexity. What it gains in flexibility, it loses in consistency.”
Maman Ibraham, principal partner at the EugeneZonda consulting firm, had a more succinct take on the genAI tool usage in financial.
“Having 20 genAI tools doesn’t make you innovative,” Ibraham said. “It makes you ungovernable.”
To mitigate risk, Apiiro recommends that each vertical calibrate its genAI coding strategies differently, based on its environment. “In retail, start with data mapping, access control audits, and early-stage static analysis to catch issues before deployment. In finance, prioritize secrets detection, dependency hygiene, and reviewing whether dormant genAI projects should be refactored or retired,” it said.
OpenAI’s o3 price plunge changes everything for vibe coders 18 Jun 2025, 5:00 am
On June 10, OpenAI slashed the list price of its flagship reasoning model, o3, by roughly 80% from $10 per million input tokens and $40 per million output tokens to $2 and $8, respectively. API resellers reacted immediately: Cursor now counts one o3 request the same as a GPT-4o call, and Windsurf lowered the “o3-reasoning” tier to a single credit as well. For Cursor users, that’s a ten-fold cost cut overnight.
Latency improved in parallel. OpenAI hasn’t published new latency metrics; third-party dashboards still see time to first token (TTFT) in the 15s to 20s range for long prompts. Thanks to fresh Nvidia GB200 clusters and a revamped scheduler that shards long prompts across more GPUs, o3 feels snappier in real use. o3 is still slower than lightweight models, but no longer coffee-break slow.
Claude 4 is fast yet sloppy
Much of the community’s oxygen has gone to Claude 4. It’s undeniably quick, and its 200k context window feels luxurious. Yet, in day-to-day coding, I, along with many Reddit and Discord posters, keep tripping over Claude’s action bias: It happily invents stubbed functions instead of real implementations, fakes unit tests, or rewrites mocks that were told to leave alone. The speed is great; the follow-through often isn’t.
o3: Careful, deliberate, and suddenly affordable
o3 behaves almost the opposite way. It thinks first, asks clarifying questions, and tends to produce code that actually compiles. Until last week, that deliberation was priced like a Jeff Bezos-style turducken superyacht. Now it’s a used Honda Civic.
Specifically, the same 4k in / 1.6k out architectural prompt fell from $0.10 to $0.02—about an 80% cut, exactly mirroring OpenAI’s official 80% price drop.
Tool calling: When ‘reasoning’ goes full Rube Goldberg
One caveat: o3 loves tool calls often too much. Windsurf users complain it “overuses unnecessary tool calls and still fails to write the code.” In my own sessions, o3 peppers the planner with diff, run tests, search, and even file-system reads more aggressively than Claude does. Claude (and smaller models) often infer the answer without explicit calls; o3 prefers to see the facts for itself. That’s great until it isn’t. Keep a finger on the kill switch. I usually tell o3 to create a series of contained subtasks so that it doesn’t try seeing the whole picture when its code time.
Some tips:
- Throttle calls: Set hard caps, e.g., “Use a maximum of 8 tool calls.”
- Demand minimal scope: Remind o3, e.g., “Touch only these two files.”
- Review diffs and commit often: As with any model, it is far from perfect.
Why reasoning matters for coding
Reasoning-heavy models excel at multi-hop constraints such as renaming a domain class, updating a database migration, fixing integration tests, and keeping the logic intact in one go. Research on chain-of-thought for code generation shows that structured reasoning improves pass-at-1 accuracy by double-digit percentages on benchmarks such as HumanEval and Mostly Basic Python Problems (MBPP). Smaller models falter after the third hop; o3 keeps more invariants in working memory, so its first draft passes more often.
But is this “real” thinking?
Apple’s recent paper “The Illusion of Thinking” argues that so-called large reasoning models (LRMs) don’t really reason; they just pattern-match longer chains of tokens. The authors show that LRMs plateau on synthetic hard-mode puzzles. That echoes what most practitioners already know: A chain of thought is powerful but not magic. Whether you label it “reasoning” or “very fancy autocomplete,” the capability boost is real, and the price drop makes that boost usable.
Under the hood: Subsidies, silicon, and scale
OpenAI can’t possibly turn a profit at $2 in / $8 out if o3 inference still costs last winter’s rates. Two forces make the math less crazy:
- Hardware leaps: Nvidia’s GB200 NVL72 promises 30× inference throughput versus equal-node H100 clusters at big energy cuts.
- Capital strategy: Oracle’s 15-year, $40B chip lease to OpenAI spreads capex over a decade, turning GPU spend into cloud-like opex.
Even so, every major vendor is in land-grab mode, effectively subsidizing floating point operations per second (FLOPS) to lock in developers before regulation and commoditization.
Rising alternatives keep the pressure on
OpenAI’s rivals smell opportunity:
- BitNet b1.58 (Microsoft Research): A 1-bit model that runs respectable code generation on CPUs, slashing infrastructure costs.
- Qwen3-235B-A22B (Alibaba): An Apache 2.0 mixture-of-experts (MoE) giant with Claude-level reasoning and only 22B parameters active per token.
BitNet does not match o3 or GPT-4o in raw capability, yet it runs on modest hardware and reminds us that progress is not limited to ever-larger models. As smaller architectures improve, they may never equal the absolute frontier, but they can reach the level most tasks demand.
Qwen continues to trail o3 in both speed and skills, but the pricing trend is clear: Advanced reasoning is becoming a low-cost commodity. Vendor subsidies might never be recouped, and without a strong lock-in, cheaper hardware and rapid open-source releases could drive the marginal cost of top-tier reasoning toward zero.
Practical workflow adjustments
- Promote o3 to your main coder and planner. The latency is now bearable, the price is sane, and the chain-of-thought pays off. What you may pay for in wait you make up in not having to rework.
- Retain a truly lightweight fallback. No one wants to wait around for reasoning for simple things like “make a branch” or “start docker.” There are many models that can do these things. Pick something light and cheap.
- Tame tool mania. Explicitly set a max tool calls rule per request, and lean on diff reviews before merging.
- Prompt economically. Even at $2 in / $8 in, sloppy prompts still burn money. Use terse system messages and reference context IDs rather than pasting full files.
- Watch latency spikes. Subsidy era or not, usage surges can reintroduce throttles. Have a backup model keyed in your IDE just in case.
- Consider alternatives to heavyweights like Cursor and Windsurf. I started making my own AI coding assistant in part so I could use different models and combinations of models. However, you can do a version of this with the more open source alternatives like Roo Code or Cline. They have their own issues, but being able to access the whole catalog of models on OpenRouter is eye opening.
The bottom line
A few weeks ago I told readers o3 was “too slow and too expensive for daily coding.” Today it’s neither. It’s still no REPL, but the brains-per-buck ratio just flipped. o3 out-codes Claude in reliability, and its cost finally lets you keep it on speed-dial without lighting your wallet on fire.
Christmas came in June and Santa Sam stuffed our stockings with subsidized FLOPS. Fire up o3, keep your prompts tight, and let the model over-think so you don’t have to.
Better together: Developing web apps with Astro and Alpine 18 Jun 2025, 5:00 am
Astro is a stable server-side platform for launching web applications. Alpine is the go-to minimalist front-end JavaScript framework. Put them together, and you have a lean, versatile stack. This article walks through developing a web application that showcases some of the best features of Astro and Alpine together.
Overview of the Astro-Alpine stack
Astro is best known as a metaframework whose purpose is to integrate reactive frameworks like React and Svelte. Alpine is also a reactive framework, but its design is so lightweight it works differently when combined with Astro, mainly by avoiding some of the formality of integration.
Astro with Alpine is an interesting stack because Astro already provides quite a bit of power to define server-side components, and Alpine’s clean syntax makes it easy to embellish different parts of the application with interactivity and API calls.
Astro includes an official Alpine plugin out of the box, so using them together is almost seamless. There’s a bit of finagling when it comes to driving an Alpine component from static-site generation (SSG) data coming from Astro, but this is a small wrinkle. Overall, it’s a good combination if you want to build something that can largely run on Astro’s SSG and then lean on Alpine for fancier interactions.
I organize the Astro-Alpine story into three development tiers, in ascending order of how heavily each tier leans on Alpine:
- Tier 1: Straight SSG with simple client-side enhancement.
- Tier 2: Dynamic SSR (server-side rendering) with client-side enhancement.
- Tier 3: Client-side rendering with API calls.
The differences among the tiers will become clearer with examples.
Setting up the example application
We’ll build a hypothetical web application called Coast Mountain Adventures. The app consists of three pages, which we’ll use to explore the three tiers of development:
- Tier 1: About page: A simple accordion on static content.
- Tier 2: Gear shops: A list of local outdoor gear shops generated by Astro and filtered by Alpine.
- Tier 3: My adventures: An API that takes a user ID and returns a JSON response containing the user’s data, rendered on the client by Alpine.
The first thing to do is install Astro, then create a new Astro application and install the Alpine integration. (Note that I also installed the Tailwind plugin for easy, responsive styling, but we won’t cover the CSS here.)
$ npm create astro@latest -- --template minimal
$ npx astro add alpinejs
$ npx astro add tailwind
Astro’s CLI is informative and easy to use.
Layout of the example app
Astro is smart about how it packages the different sections of your app. Our framing material (the layout) is simple, just a title and some links. Astro will bundle them up and ship them without any JavaScript:
---
import './src/styles/global.css';
---
Astro Alpine
There’s also some styling and a CSS import, which we are skipping over. Notice the
; element—that’s where Astro will put the pages that use this layout. (Check the documentation for more about Astro layouts.) Astro also handles routing the URLs to the right files on disk in the /src/pages
directory.
The About page
Our About page is SSR with client-side enhancements. It has three sections: a main section and two subsections. We probably wouldn’t do this in real life because we wouldn’t want to ever hide this content, but for this tutorial, we’ll make the two subsections accordion panels. Clicking them will show or hide their bodies.
---
import Layout from '../layouts/Layout.astro';
---
About Us
We are a consortium of outdoor enthusiasts and locally owned gear shops and exchanges, dedicated to the truth that when nature and people come together, Good Things happen.
Our Mission
{/* Add the icon span */}
↓ {/* Down arrow character */}
To connect people with nature and provide access to quality outdoor gear, experiences and curated resources.
Our Values
↓
- Community
- Sustainability
- Adventure
Except for using the Astro layout, there is nothing Astro-specific to this section; it’s just HTML with a few Alpine directives sprinkled around. The three types of directives used are:
x-data
: Defines the data object for the Alpine component.x-on:click
: Defines an onclick handler.x-show
: Conditionally shows or hides the element.
Those are all we need for our accordions, plus a touch of CSS for the arrow icons. Here’s how the page looks so far:
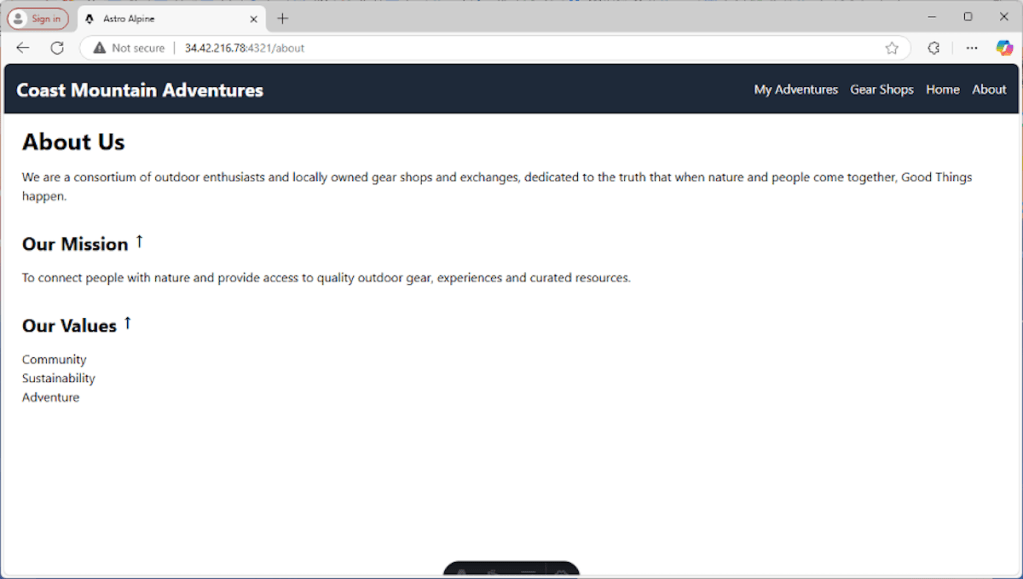
Matthew Tyson
See my introduction to Alpine for more about working with Alpine.
The gear shop page
The gear-shop page is mainly server-side data with client-side filtering. For this page, we want to take a chunk of data that is available on the back end and render it in such a way that the client can filter it. That means we’ll want to server-side render the data with Astro and create an Alpine component that can consume it, providing the dynamic UI interaction.
First up is the page itself:
---
// src/pages/gear-shops.astro
import Layout from '../layouts/Layout.astro';
import GearShopList from '../components/GearShopList.astro';
const gearShops = [
{ name: "Adventure Outfitters", category: "Hiking" },
{ name: "Peak Performance Gear", category: "Climbing" },
{ name: "River Rat Rentals", category: "Kayaking" },
{ name: "The Trailhead", category: "Hiking" },
{ name: "Vertical Ventures", category: "Climbing" }
];
---
Local Gear Shops
We’ll see the GearShopList
component in just a minute. The data is in the gearShops
variable. This could come from a database or an external API. It could come from a local filesystem or Astro’s built-in content collections. The point is, this data is produced on the server. The trick now is to make it available as live JSON on the client, where Alpine can use it.
The first step is to turn the data into a string on the shops
property of the GearShopList
component. This means Astro can serialize it when it sends the view over to the browser. (In essence, the JSON.stringify(gearShops)
will be inlined into the HTML document.)
Here’s a look at GearShopList
:
---
const { shops, initialCount } = Astro.props; // Receive the 'shops' prop
if (!shops) {
throw new Error('GearShopList component requires a "shops" prop that is an array.');
}
---
-
()
This is all fairly self-explanatory except for the x-data
attribute:
The purpose of this is clear enough: We want a JavaScript object that has a filter
field and a shops
field, where shops
has the live JavaScript object we created back on the server. How exactly to do that, syntax-wise, is the source of all the extra characters. The key to making sense of that x-data
value is the “{``}
” syntax—enclosing curly braces with two backticks inside—which resolves to an empty string.
This means the x-data
field ultimately will be a string-literal object with the shops
variable interpolated as JSON, and Alpine will consume all that and turn it into a live object to drive the component. (There is an Astro-Alpine demo app on GitHub that uses a counter component that works similarly to our shops
variable. Looking at it should help clarify how this part of the app works.)
Besides the x-data
attribute, we have an x-model
on the select that binds the selection value to the filter variable. The x-for
lets us iterate over the shops, and we use some functional decision-making to only x-show
the items that match the filter.
Here’s how the gear shop page looks:
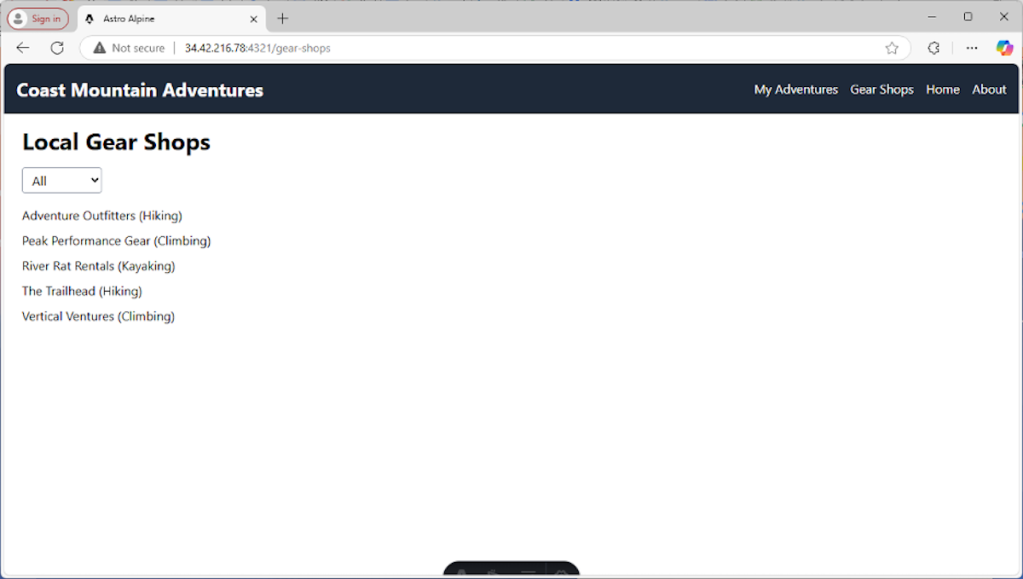
Matthew Tyson
The adventures page
This page is composed of client-side rendering with API calls—so in this case, Alpine is in charge. We will disable pre-rendering, so Astro doesn’t do any SSG:
---
import Layout from '../layouts/Layout.astro';
export const prerender = false; // Important: Disable prerendering for this page
---
My Adventures
Loading adventures...
{/*... other adventure details... */}
This page really shows off Alpine’s versatility. Notice how the x-data
attribute includes both the data
property (adventures
) and a method that does fetching (fetchAdventures()
). This is one of my favorite parts of Alpine; it’s just so clean and powerful.
When x-init
is called upon component initiation, it calls that fetchAdventures()
function and that will make the call to our server and populate adventures. It’s all nicely contained in a small area where the data, behavior, and view are logically related.
We then use x-if
to conditionally render a loading message or the actual list, based on adventures.length
.
The adventures API
We also need a back-end endpoint to provide the adventures JSON for that fetch call. Here it is:
export async function GET() {
const adventures = [
{ id: 1, title: "Hiking Trip to Mount Hood", date: "2024-08-15" },
{ id: 2, title: "Kayaking Adventure on the Rogue River", date: "2024-09-22" },
//... more adventures
];
return new Response(JSON.stringify(adventures), {
status: 200,
headers: {
'Content-Type': 'application/json'
}
});
}
Overall, the client-side-with-API-call using Alpine and Astro is smooth sailing. If things were to get more complex, you would probably want to rely on a client-side store. At any rate, this stack gets the job done with minimal fuss.
Here’s our adventures page:
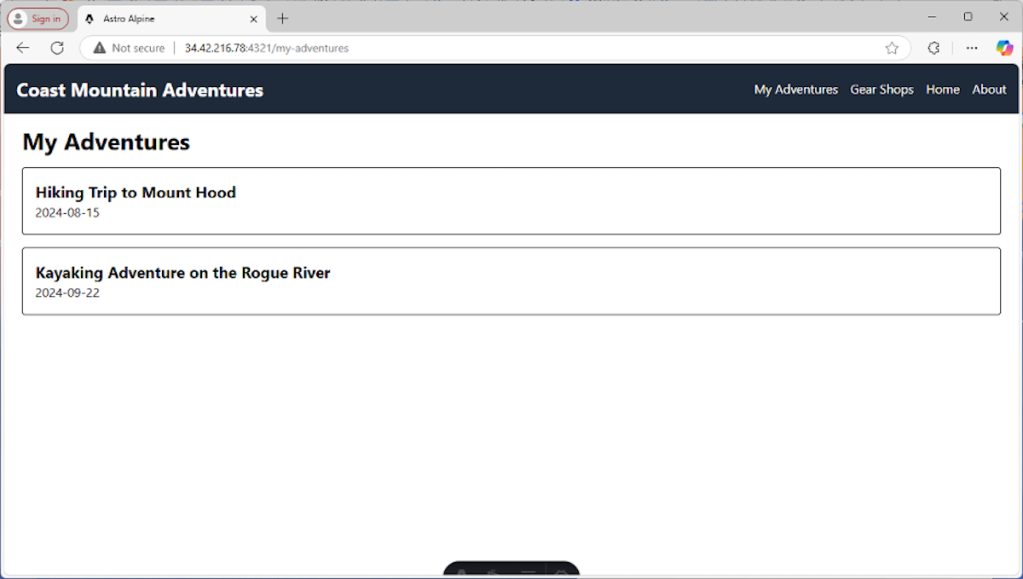
Matthew Tyson
Conclusion
For software developers who enjoy building software, it’s a happy day when you find tools that work well and yield that ineffable quality of developer experience. Both Astro and Alpine have that quality. Using them together is also very gratifying. The only hurdle is sorting out how to pass data to Alpine during SSR.
Get started with Python type hints 18 Jun 2025, 5:00 am
Python is best thought of as a dynamic but strongly typed language. Types aren’t associated with the names of things, but with the things themselves.
This makes Python flexible and convenient for developers because you don’t have to rigorously define and track variable types if you’re just throwing together a quick-and-dirty script. But for bigger projects, especially libraries used by third parties, it helps to know which object types are associated with which variables.
For some time now, Python developers have been able to “annotate” names with type information. With Python 3.5, type hints officially became part of the language (see PEP 484). Using a linter or code-checking tool, developers can check the consistency of variables and their types across a codebase. We can perform static analysis on code that would previously have been difficult or impossible. All this is done ahead of time, before the code runs.
In this article, we’ll explore some basic examples of Python type hinting, including the lazy evaluation of annotations, now the default in Python 3.14. But first, we’ll clear up a common misunderstanding about what type hinting is and isn’t used for.
How Python uses type hints (it doesn’t)
A key misconception about Python type hints is how they’re used. Python type hints are not used at runtime, at least not ordinarily. In fact, when your program runs, all the type information you’ve provided is ignored (although it is preserved in some form; more on this later). Python type hints are used ahead of time, by the type-checking system; for instance, in your editor or IDE. In other words, Python’s type hints are for the developer, not the runtime.
This may sound counterintuitive, especially if you’ve worked with languages where type declarations were not optional. But Python’s development team has gone out of its way to make clear that type hints aren’t a prelude to the core Python language becoming statically typed. They’re a way for developers to add metadata to a codebase, making it easier to perform static analysis during development.
Some have speculated that Python type hinting could in time give rise to a fork of the language that is statically typed, perhaps as a way to make Python faster. In some ways, this fork has already arrived: Cython uses type hints (although mostly its own peculiar breed of them) to generate C code from Python, and the mypyc
project uses Python’s native type hinting to do the same. Additionally, projects like Codon and Mojo use type declarations to create speedier, alternative dialects of Python.
But these projects are more properly thought of as complements to the core Python language, rather than signs of where Python is headed. The main purpose of type hinting in Python is to give developers a way to make their code as self-describing as possible, both for their own benefit and that of other developers.
The syntax of Python type hints
Type hints in Python involve a colon and a type declaration after the first invocation of a name in a namespace. Here’s an example:
name: str
age: int
name = input("Your name?")
age = int(input("Your age?"))
# alternatively, and more simply:
name: str = input("Your name?")
age: int = int(input("Your age?"))
The first declarations of name
and age
with type hints ensure that any future use of those names in that namespace will be checked against those types. For instance, this code would be invalid:
name: int
age: int
name = input("Your name?")
age = int(input("Your age?"))
Because we declared name
as an int
already, and input
by default returns a string, the type checker would complain. The code would still run, however, since type hints are not used at runtime. Outside of the mis-hinted types, the code doesn’t do anything wrong.
Python type-checking systems will, whenever possible, try to infer types. For instance, let’s say we used the following code without the previous type declarations:
name = input("Your name?")
age = int(input("Your age?"))
In that case, the type checker would be able to infer that name
is a string (since input()
doesn’t return anything else), and that age
is an int
(since int()
doesn’t return anything else). But the best results come from hinting each variable explicitly, since it isn’t always possible to make these inferences.
Type hinting Python functions
Python functions can also be type hinted, so that the values they accept and return are documented ahead of time. Consider the following code:
greeting = "Hello, {}, you're {} years old"
def greet(user, age):
return greeting.format(user, age)
name = input("Your name?")
age = int(input("How old are you?"))
print(greet(name, age))
One ambiguity with this code is that greet()
could in theory accept any types for user
and age
, and could return any type. We could disambiguate that confusion using type hints:
greeting = "Hello, {}, you're {} years old"
def greet(user: str, age: int) -> str:
return greeting.format(user, age)
name = input("Your name?")
age = int(input("How old are you?"))
print(greet(name, age))
Given these type hints for greet()
, your editor could tell you ahead of time which types greet()
will accept when you insert a call to it into your code.
Again, sometimes Python can automatically infer what types are returned from a function. But if you plan on using type hinting with a function, it’s best to hint everything about it—what types it takes in as well as what types it returns.
Type hinting container objects
Because objects like lists, dictionaries, and tuples contain other objects, we sometimes want to type hint them to indicate what kinds of objects they contain. Previously, Python required using the typing
module to provide tools for describing such types. As of Python 3.9, they can be done natively in Python:
dict_of_users: dict[int,str] = {
1: "Jerome",
2: "Lewis"
}
list_of_users: list[str] = [
"Jerome", "Lewis"
]
Dictionaries are made of keys and values, which can be of different types. You can describe the types for a dictionary by hinting with dict[
. And you can describe the object type for a list with a hint in the format of list[
.
Optional and Union types
Some objects contain one of a couple of different types of objects. In these cases, you can use Union
or Optional
. Use Union
to indicate that an object can be one of several types. Use Optional
to indicate that an object is either one given type or None
. For example:
from typing import Dict, Optional, Union
dict_of_users: Dict[int, Union[int,str]] = {
1: "Jerome",
2: "Lewis",
3: 32
}
user_id: Optional[int]
user_id = None # valid
user_id = 3 # also vald
user_id = "Hello" # not valid!
In this case, we have a dictionary that takes int
s as keys, but either int
s or str
s as values. The user_id
variable (which we could use to compare against the dictionary’s keys) can be an int
or None
(“no valid user”), but not a str
.
In Python 3.10 and higher, Union
types can be hinted using the pipe character as follows, with no imports needed:
dict_of_users: dict[int, int|str] = {
1: "Jerome",
2: "Lewis",
3: 32
}
Also note that Optional
can be hinted as a Union
with None
; for example, int|None
instead of Optional[int]
. This saves you from needing to import Optional
if you’d rather keep things simple.
Type hinting and classes
To provide type hints for classes, just reference their names the same as you would any other type:
class User:
def __init__(self, name: str):
self.name = name
users: dict[int, User] = {
1: User("Serdar"),
2: User("Davis")
}
def inspect_user(user: User) -> None:
print (user.name)
user1 = users[1]
inspect_user(user1)
Note that inspect_user()
has a return type of None
because it only prints output and does not return anything. (We’d normally make such a thing into a method for the class, but it’s broken out separately here for illustration.)
Also note we have the __init__
method type-hinted; name
is hinted as str
. We do not need to hint self
; the default behavior with Python linting is to assume self
is the class. If you need an explicit self
hint for the class that refers to whatever class is in context, and you’re using Python 3.11 or later, you can import Self
from the typing
module and use that as a hint.
Deferred evaluation of annotations
When using type hints for custom objects, we sometimes need to provide a type hint for an object that hasn’t yet been defined. For some time, a common way to do this was to provide the object name as a string hint. This is a deferred annotation; it allows the object reference to be resolved lazily:
class User:
def __init__(self, name: str, address: "Address"):
self.name = name
self.address = address
# ^ because let's say for some reason we must have
# an address for each user
class Address:
def __init__(self, owner: User, address_line: str):
self.owner = owner
self.address_line = address_line
This approach is useful if you have objects with interdependencies, as in the above example. There is probably a more elegant way to untangle it, but at least you can provide ahead-of-time hints in the same namespace simply by providing the name of the object.
However, a better way to do this is to use a feature called deferred evaluation of annotations. You can use a special import to change the way annotations are resolved at the module level:
from __future__ import annotations
class User:
def __init__(self, name: str, address: Address):
self.name = name
self.address = address
# ^ because let's say for some reason we must have
# an address for each user
class Address:
def __init__(self, owner: User, address_line: str):
self.owner = owner
self.address_line = address_line
When you add from __future__ import annotations
at the top of a module, annotations are now resolved lazily for that module—just as if they were string hints, but the actual object is referred to instead of just its name. This allows linters to resolve things far more powerfully and completely, which is why it’s the recommended solution for this problem. You can still use string hints where they’re expected, but they should be phased out.
As of Python 3.14, the lazy evaluation of annotations is the default behavior. You still can use the above code without the __future__
import but in the future, it may generate deprecation warnings.
Malicious PyPI package targets Chimera users to steal AWS tokens, CI/CD secrets 17 Jun 2025, 8:38 am
A malicious Python package posing as a harmless add-on for the Chimera sandbox environment, an integrated machine learning experimentation and development tool, is helping threat actors steal sensitive corporate credentials.
According to new research findings from software supply chain and DevOps company JFrog, the package “chimera-sandbox-extensions”, recently uploaded to the popular PyPI repository, contains a stealthy, multi-stage info-stealer.
Amazon Bedrock faces revamp pressure amid rising enterprise demands 17 Jun 2025, 5:33 am
Amazon’s flagship generative AI platform, Bedrock, could be in for a significant overhaul as customer expectations evolve and competition intensifies between the three major cloud providers — AWS, Microsoft Azure, and Google Cloud.
Analysts suggest that growing customer demand for updated model offerings and tooling inside Bedrock may push AWS to rethink its strategy around its generative AI offerings, potentially signaling a major shift in its generative AI roadmap.
Enterprise customers have long voiced frustration over the absence of OpenAI’s popular large language models (LLMs) and the lack of a cohesive agent-building framework within Bedrock, said Eric Miller, VP of data and AI at ClearScale, a cloud consultancy and AWS partner, about organizations it works with.
The problem around the unavailability of OpenAI’s LLMs is further compounded due to cost-related challenges that further complicate adoption, according to Derek Ashmore, AI enablement principal at Asperitas Consulting, a cloud consultancy firm and AWS partner.
“As enterprises must call OpenAI’s APIs separately to access its LLMs, they run into data egress fees when transferring results from OpenAI’s service back into AWS. There is also the challenge of additional integration overhead for managing external API calls outside of Bedrock’s unified environment,” Ashmore said.
Similarly, The Futurum Group’s lead of the CIO practice, Dion Hinchcliffe, pointed out that the act of calling external APIs introduces latency as well as security concerns.
“CIOs I talk with find this fragmentation increasingly untenable for production workloads,” Hinchcliffe said.
Amazon Bedrock’s problems with perception and tooling
Amazon Bedrock is grappling with a perception and tooling challenge that’s increasingly hard to ignore for enterprise customers.
“Enterprises are increasingly looking for more than just raw access to foundation models — they want frameworks that simplify building applications. While Bedrock offers a strong selection of models, it lacks higher-level tools for agent orchestration, retrieval-augmented generation (RAG), and workflow automation — leading to a disjointed developer experience,” said Ashmore.
In contrast, Microsoft Azure and Google Cloud have invested heavily in features like Copilot Studio, Azure AI Foundry, and Vertex AI Agent Builder, which provide these capabilities out of the box, Ashmore added.
The lack of tooling and the changing perception of Bedrock, according to Miller, is in the service’s origin story and how the market views it: “It has really set itself apart from rival offerings as more of a model marketplace or a nexus of models behind a common API interface rather than an orchestrating platform.”
Late to the open-source agent development framework party?
Another challenge that AWS faces is in the open-source agent development framework category, whose usage has become a significant trend within the developer community. This is evident by the growing adoption of Crew AI, Hugging Face, Microsoft’s AutoGen, and Google’s Agent Development Kit (ADK).
While the cloud services provider did release Strands Agents, an open-source AI Agents SDK last month, Google’s ADK and Microsoft’s AutoGen, which were released earlier, have “already set a high bar for open source, developer-centric software for agent creation and is more widely adopted,” said Igor Beninca, data science manager at Indicium — a AI and data consultancy firm.
Additionally, Strands Agents, unlike ADK’s and AutoGen’s integration into Vertex AI and Azure OpenAI and Azure AI Foundry, respectively, is not natively integrated into Bedrock, although it uses a few Bedrock tools — a growing demand among developers and CIOs, Hinchcliffe pointed out.
“Customers say that Strand Agents feel stranded as they are not integrated into Bedrock, forcing them to stitch workflows manually. This breaks AWS’s promise to customers that Bedrock is a managed, secure, and scalable generative AI service,” Hincliffe said.
However, AWS seems to be taking cognizance of customer and partner feedback on Bedrock.
The cloud services provider, according to Hinchcliffe, has been “quietly preparing a major uplift of Bedrock” focused on making the service agent-native, RAG-friendly, and finally elastic in pricing.
“Think managed agent hosting, deeper memory or state support, and tunable orchestration across model endpoints. The goal is to move from ‘just inference’ to ‘intelligent automation,’ especially for large enterprises already deploying multi-agent systems via open source on raw EC2,” Hinchcliffe said.
The Information was the first to report the possible revamp of Bedrock.
Notably, AWS and its leadership have often said that its offerings are always based on and improved by customer feedback.
But is a major revamp really required?
Experts are divided on whether a major revamp of Bedrock is currently necessary. The debate centers on enterprises voicing issues around trying to use Bedrock in conjunction with an open-source agent development framework, which rival offerings, such as Vertex AI, don’t pose.
“Currently, if customers want to use frameworks like AutoGen or CrewAI on AWS, they have to run them manually on EC2 instances — a bit of an anti-pattern for customers who seek to operate truly cloud native or desire to use a unified development environment,” Miller said.
However, Miller pointed out that customers are not, in all likelihood, asking for a major revamp but a new separate managed service that allows them to run open-source agent development frameworks on AWS without managing their own EC2 infrastructure.
“They (customers) don’t want to size, deploy, manage, and maintain EC2 instances to run this software…they just want to turn something on inside the AWS Console, and start building via the console or SDK, and then pay for it consumption-based as they go, while AWS manages the service uptime, etc.,” Miller said.
Explaining further, Miller said that AWS is at a turning point in its generative AI strategy, comparing the potential revamp of Bedrock to the shift from Elastic Container Service (ECS) to Elastic Kubernetes Service (EKS) — when AWS adapted to customer demand for Kubernetes.
“If AWS launches a turnkey, cloud-native service for agentic frameworks today, it could be the EKS moment for Bedrock — delivering exactly what customers are asking for and setting a new standard in the space,” Miller added.
A call for a clearer platform strategy
In contrast, Asperitas Consulting’s Ashmore said that “there is a need for AWS” to clarify its platform strategy as well as remain competitive with its rivals.
“Right now, the relationship between Strand Agents, Bedrock, Amazon Q, and SageMaker isn’t well-defined. A revamp could bring these together into a more cohesive, developer-friendly offering that simplifies how customers build and scale agent-based applications on AWS,” Ashmore added.
In addition, Ashmore pointed out that AWS may need to act fast as enterprises are already looking at alternatives that provide the capability to access an open-source agent development framework within a unified development platform.
For instance, ClearScale has built an offering, ClearScale GenAI workbench, which is a wrapper around Bedrock that adds agentic framework capabilities, specifically AutoGen, and many other features that make building agents with Bedrock relatively easy.
Navigating the rising costs of AI inferencing 17 Jun 2025, 5:00 am
In 2025, the worldwide expenditure on infrastructure as a service and platform as a service (IaaS and PaaS) reached $90.9 billion, a 21% rise from the previous year, according to Canalys. From I’m seeing, this surge is primarily driven by companies migrating their workloads to the cloud and adopting AI, which relies heavily on compute resources. Yet as businesses eagerly embrace these technologies, they are also encountering obstacles that could hinder their strategic use of AI.
Transitioning AI from research to large-scale deployment poses a challenge in distinguishing between the costs associated with training models and those linked to inferring them. Rachel Brindley, senior director at Canalys, notes that, although training usually involves a one-time investment, inferencing comes with expenses that may vary considerably over time. Enterprises are increasingly concerned about the cost-effectiveness of inference services as their AI projects move towards implementation. It is crucial to pay attention to this, as costs can quickly add up and create pressure for companies.
Today’s pricing plans for inferencing services are based on usage metrics, such as tokens or API calls. As a result, companies may find it difficult to predict their costs. This unpredictability could lead businesses to scale back the sophistication of their AI models, restrict deployment to critical situations, or even opt out of inferencing services altogether. Such cautious strategies might hinder the overall advancement of AI by constraining organizations to less cutting-edge approaches.
The effects of busted budgets
The concern about inference-related expenses is justified, as several businesses have experienced the consequences of overestimating their requirements and incurring high bills as a result. A notable example is 37signals, which operates the Basecamp project management tool and faced a cloud bill surpassing $3 million. This unexpected discovery led the company to transition its IT infrastructure management from the cloud to on premises.
Organizations are increasingly aware of the risks associated with utilizing cloud services like never before. Gartner has cautioned that companies venturing into AI adoption could encounter cost estimation discrepancies ranging from 500% to 1,000%. These may stem from hikes in vendor prices, overlooked expenses, and mismanagement of AI resources. As businesses explore the potential of AI technologies, miscalculating their budgets is a significant risk to innovation and progress.
Exploring different hosting options
Many organizations are now reassessing their approaches to the cloud. As companies increasingly depend on public cloud services, such as Amazon Web Services (AWS), Microsoft Azure, and Google Cloud, some are turning towards specialized hosting providers or colocation services. These options offer a pricing structure that could improve resource handling, enabling businesses to enhance their AI applications without worrying about unexpected costs.
Currently, the top cloud services companies hold a significant market position, with more than 65% of customer expenditure under their control. However, AWS has experienced a decrease in growth, from 17% in the most recent quarter compared to 19% in the preceding one. On the other hand, Microsoft and Google have maintained growth rates surpassing 30%. Businesses are looking for cost-effectiveness and tailored solutions offered by specialized providers.
Cloud service providers are aware of the challenges associated with inference expenses and are actively investigating methods to enhance effectiveness and lower service charges. According to Canalys, specialized expertise in AI tasks might be crucial in alleviating the burden of inferencing expenses by integrating tailored hardware accelerators alongside GPUs, optimizing efficiency and reducing costs.
Despite these efforts to implement AI on a large scale in public cloud environments, there are still doubts about its long-term sustainability. Alastair Edwards, chief analyst at Canalys, has highlighted the risks of using AI in the cloud, noting that costs could become unmanageable as organizations expand their AI projects. This poses a challenge for businesses seeking to ensure the long-term success of their AI initiatives.
Practical steps to controlling inferencing costs
In addressing the challenges faced by businesses today, it’s essential to take a proactive stance towards controlling inferencing expenses. I recommend considering the following approaches:
- Use tools that offer real-time insights into how resources are being used and how money is being spent. By monitoring cloud usage patterns, organizations can make informed choices on where to expand and where to save.
- Perform cost estimations to predict costs depending on different usage trends, aiding in forecasting expenses and preventing budget overages.
- Select the pricing model wisely by comparing options offered by cloud providers. Usage-based pricing may not be the best choice in all cases; fixed pricing could be more suitable for specific organizational requirements.
- Consider a combination of public and private cloud resources. A hybrid cloud can enhance flexibility and optimize costs effectively.
Working together with cloud service providers can help you uncover ways to manage costs effectively and efficiently. Providers often offer customized solutions designed to address industry-specific challenges.
The path to successfully integrating AI into the business world is full of obstacles, especially when it comes to controlling the expenses of making inferences in cloud setups. As businesses increasingly incorporate AI solutions into their operations, it becomes crucial to prioritize cost-effectiveness and practices. Being aware and taking steps to address these hurdles are important for companies to fully leverage the power of AI and spur creativity in their industries. Don’t wait for an unexpectedly high bill before you decide to act.
A developer’s guide to AI protocols: MCP, A2A, and ACP 17 Jun 2025, 5:00 am
Unlike traditional AI models that respond to single prompts (like ChatGPT’s basic Q&A mode), AI agents can plan, reason, and execute multi-step tasks by interacting with tools, data sources, APIs, or even other agents.
Sounds abstract? That’s because it is. While most might agree with this definition or expectation for what agentic AI can do, it is so theoretical that many AI agents available today wouldn’t make the grade.
As my colleague Sean Falconer noted recently, AI agents are in a “pre-standardization phase.” While we might broadly agree on what they should or could do, today’s AI agents lack the interoperability they’ll need to not just do something, but actually do work that matters.
Think about how many data systems you or your applications need to access on a daily basis, such as Salesforce, Wiki pages, or other CRMs. If those systems aren’t currently integrated or they lack compatible data models, you’ve just added more work to your schedule (or lost time spent waiting). Without standardized communication for AI agents, we’re just building a new type of data silo.
No matter how the industry changes, having the expertise to turn the potential of AI research into production systems and business results will set you apart. I’ll break down three open protocols that are emerging in the agent ecosystem and explain how they could help you build useful AI agents—i.e., agents that are viable, sustainable solutions for complex, real-world problems.
The current state of AI agent development
Before we get into AI protocols, let’s review a practical example. Imagine we’re interested in learning more about business revenue. We could ask the agent a simple question by using this prompt:
Give me a prediction for Q3 revenue for our cloud product.
From a software engineering perspective, the agentic program uses its AI models to interpret this input and autonomously build a plan of execution toward the desired goal. How it accomplishes that goal depends entirely on the list of tools it has access to.
When our agent awakens, it will first search for the tools under its /tools directory. This directory will have guiding files to assess what is within its capabilities. For example:
/tools/list
/Planner
/GenSQL
/ExecSQL
/Judge
You can also look at it based on this diagram:
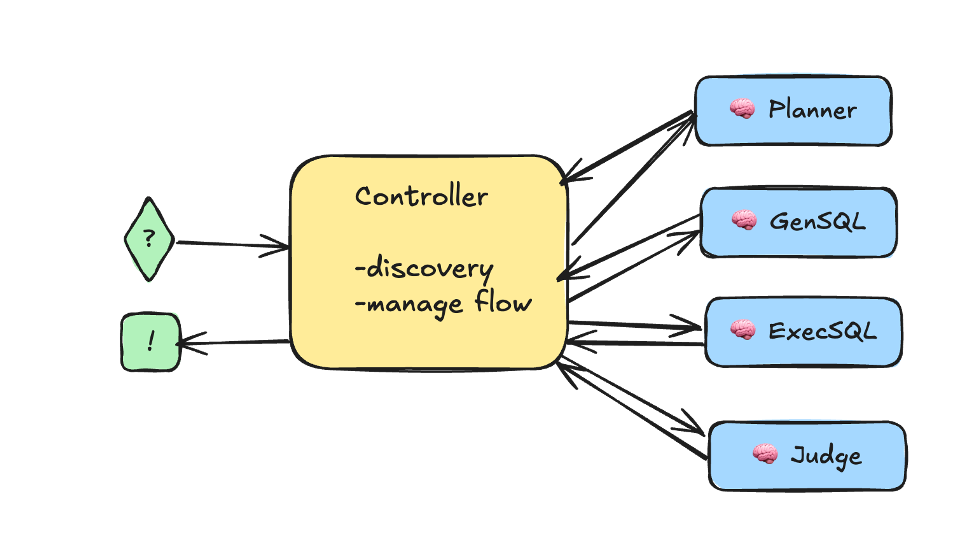
Confluent
The main agent receiving the prompt acts as a controller. The controller has discovery and management capabilities and is responsible for communicating directly with its tools and other agents. This works in five fundamental steps:
- The controller calls on the planning agent.
- The planning agent returns an execution plan.
- The judge reviews the execution plan.
- The controller leverages GenSQL and ExecSQL to execute the plan.
- The judge reviews the final plan and provides feedback to determine if the plan needs to be revised and rerun.
As you can imagine, there are multiple events and messages between the controller and the rest of the agents. This is what we will refer to as AI agent communication.
Budding protocols for AI agent communication
A battle is raging in the industry over the right way to standardize agent communication. How do we make it easier for AI agents to access tools or data, communicate with other agents, or process human interactions?
Today, we have Model Context Protocol (MCP), Agent2Agent (A2A) protocol, and Agent Communication Protocol (ACP). Let’s take a look at how these AI agent communication protocols work.
Model Context Protocol
Model Context Protocol (MCP), created by Anthropic, was designed to standardize how AI agents and models manage, share, and utilize context across tasks, tools, and multi-step reasoning. Its client-server architecture treats the AI applications as clients that request information from the server, which provides access to external resources.
Let’s assume all the data is stored in Apache Kafka topics. We can build a dedicated Kafka MCP server, and Claude, Anthropic’s AI model, can act as our MCP client.
In this example on GitHub, authored by Athavan Kanapuli, Akan asks Claude to connect to his Kafka broker and list all the topics it contains. With MCP, Akan’s client application doesn’t need to know how to access the Kafka broker. Behind the scenes, his client sends the request to the server, which takes care of translating the request and running the relevant Kafka function.
In Akan’s case, there were no available topics. The client then asks if Akan would like to create a topic with a dedicated number of partitions and replication. Just like with Akan’s first request, the client doesn’t require access to information on how to create or configure Kafka topics and partitions. From here, Akan asks the agent to create a “countries” topic and later describe the Kafka topic.
For this to work, you need to define what the server can do. In Athavan Kanapuli’s Akan project, the code is in the handler.go file. This file holds the list of functions the server can handle and execute on. Here is the CreateTopic
example:
// CreateTopic creates a new Kafka topic
// Optional parameters that can be passed via FuncArgs are:
// - NumPartitions: number of partitions for the topic
// - ReplicationFactor: replication factor for the topic
func (k *KafkaHandler) CreateTopic(ctx context.Context, req Request) (*mcp_golang.ToolResponse, error) {
if err := ctx.Err(); err != nil {
return nil, err
}
if err := k.Client.CreateTopic(req.Topic, req.NumPartitions, req.ReplicationFactor); err != nil {
return nil, err
}
return mcp_golang.NewToolResponse(mcp_golang.NewTextContent(fmt.Sprintf("Topic %s is created", req.Topic))), nil
}
While this example uses Apache Kafka, a widely adopted open-source technology, Anthropic generalizes the method and defines hosts. Hosts are the large language model (LLM) applications that initiate connections. Every host can have multiple clients, as described in Anthropic’s MCP architecture diagram:
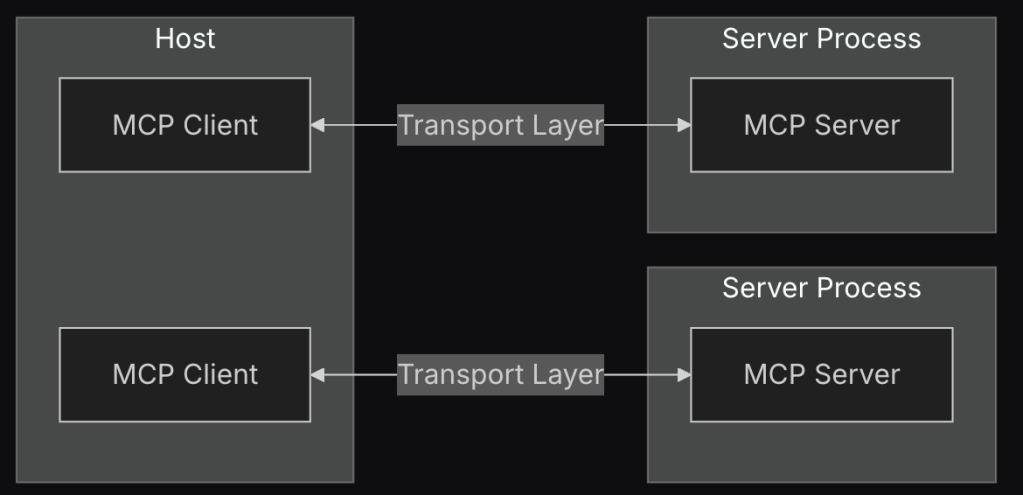
Anthropic
An MCP server for a database will have all the database functionalities exposed through a similar handler. However, if you want to become more sophisticated, you can define existing prompt templates dedicated to your service.
For example, in a healthcare database, you could have dedicated functions for patient health data. This simplifies the experience and provides prompt guardrails to protect sensitive and private patient information while ensuring accurate results. There is much more to learn, and you can dive deeper into MCP here.
Agent2Agent protocol
The Agent2Agent (A2A) protocol, invented by Google, allows AI agents to communicate, collaborate, and coordinate directly with each other to solve complex tasks without frameworks or vendor lock-in. A2A is related to Google’s Agent Development Kit (ADK) but is a distinct component and not part of the ADK package.
A2A results in opaque communication between agentic applications. That means interacting agents don’t have to expose or coordinate their internal architecture or logic to exchange information. This gives different teams and organizations the freedom to build and connect agents without adding new constraints.
In practice, A2A requires that agents are described by metadata in identity files known as agent cards. A2A clients send requests as structured messages to A2A servers to consume, with real-time updates for long-running tasks. You can explore the core concepts in Google’s A2A GitHub repo.
One useful example of A2A is this healthcare use case, where a provider’s agents use the A2A protocol to communicate with another provider in a different region. The agents must ensure data encryption, authorization (OAuth/JWT), and asynchronous transfer of structured health data with Kafka.
Again, check out the A2A GitHub repo if you’d like to learn more.
Agent Communication Protocol
The Agent Communication Protocol (ACP), invented by IBM, is an open protocol for communication between AI agents, applications, and humans. According to IBM:
In ACP, an agent is a software service that communicates through multimodal messages, primarily driven by natural language. The protocol is agnostic to how agents function internally, specifying only the minimum assumptions necessary for smooth interoperability.
If you take a look at the core concepts defined in the ACP GitHub repo, you’ll notice that ACP and A2A are similar. Both have been created to eliminate agent vendor lock-in, speed up development, and use metadata to make it easy to discover community-built agents regardless of the implementation details. There is one crucial difference: ACP enables communication for agents by leveraging IBM’s BeeAI open-source framework, while A2A helps agents from different frameworks communicate.
Let’s take a deeper look at the BeeAI framework to understand its dependencies. As of now, the BeeAI project has three core components:
- BeeAI platform – To discover, run, and compose AI agents;
- BeeAI framework – For building agents in Python or TypeScript;
- Agent Communication Protocol – For agent-to-agent communication.
What’s next in agentic AI?
At a high level, each of these communication protocols tackles a slightly different challenge for building autonomous AI agents:
- MCP from Anthropic connects agents to tools and data.
- A2A from Google standardizes agent-to-agent collaboration.
- ACP from IBM focuses on BeeAI agent collaboration.
If you’re interested in seeing MCP in action, check out this demo on querying Kafka topics with natural language. Both Google and IBM released their agent communication protocols only recently in response to Anthropic’s successful MCP project. I’m eager to continue this learning journey with you and see how their adoption and evolution progress.
As the world of agentic AI continues to expand, I recommend that you prioritize learning and adopting protocols, tools, and approaches that save you time and effort. The more adaptable and sustainable your AI agents are, the more you can focus on refining them to solve problems with real-world impact.
Adi Polak is director of advocacy and developer experience engineering at Confluent.
—
Generative AI Insights provides a venue for technology leaders—including vendors and other outside contributors—to explore and discuss the challenges and opportunities of generative artificial intelligence. The selection is wide-ranging, from technology deep dives to case studies to expert opinion, but also subjective, based on our judgment of which topics and treatments will best serve InfoWorld’s technically sophisticated audience. InfoWorld does not accept marketing collateral for publication and reserves the right to edit all contributed content. Contact doug_dineley@foundryco.com.
Design a better customer experience with agentic AI 17 Jun 2025, 5:00 am
Whereas large language models (LLMs) attracted consumers well before enterprise users, SaaS platforms are driving the use of embedded AI agents to improve employee experience and productivity. AI agents are changing the future of work by helping HR leaders recruit personnel, marketers personalize ad campaigns, and IT service desk professionals respond to helpdesk tickets.
Building on this trend, the question is when—not if—embedded AI agents will become the new de facto standard for customer experience (CX). Instead of clunky user interfaces, complex search tools, and long data entry forms, businesses will simplify customer interactions with AI agents that know their preferences.
“Businesses seeking AI-driven value in customer experience will deploy specialized AI agents with domain expertise in product lines, inventory, pricing, delivery, and legal constraints,” says John Kim, CEO of Sendbird. “This shift is already transforming industries like retail, where AI enhances shopping through personalization and proactive service. In the future, consumers will have personal AI assistants—or multiple agents for finance, entertainment, healthcare, and travel.”
Start with the most boring, repetitive tasks
Some brands that pushed AI-enabled CX capabilities too early ran into issues impacting customers and their brands. Many organizations are taking a cautious approach to using AI agents to enhance customer experience, focusing their efforts on key prerequisites such as AI governance, data quality, and testing.
Early opportunities may be found in identifying boring use cases with a narrow scope, and with experiences that frustrate customers and occur at scale.
“The biggest impact that genAI and agentic AI can create is automating the most tedious, repetitive CX micro-workflows,” says Dave Singer, global VP at Verint. “A range of CX tasks, such as asking the right questions at the start of a customer interaction for context, searching for answers to customer questions, and conducting post-call wrap-up, can be automated with specialized AI-powered bots to drive stronger, faster business outcomes. As a result, agents have more capacity, CX is enhanced, and either cost savings, revenue generation, or both are increased.”
One opportunity is to consider the documentation and tools customers use to learn, install, and troubleshoot the product. Using an AI agent to answer questions can be a faster and easier experience than asking customers to find and read through many pages of documentation.
“Think about the ‘watering holes’ customers go to in their journey to use your product, like product help pages, user wikis, and online communities, and how genAI and LLMs can make those better and improve the customer’s experience,” says Jon Kennedy, CTO of Quickbase. “Make your solutions easier to use and more effective with a library of pre-built templates that can be accessed with a few prompts, all in the service of customers by industry, role, or other segments. Think about the ongoing education of those customers and how AI can help lead them to the next milestone to improve the customer journey, using the combined experiences built up in your customer community.”
Deon Nicholas, founder and president of Forethought, says to look beyond surfacing information faster and find easy ways to handle simple customer tasks. He says, “One of the easiest user experiences to develop with LLMs is a chatbot that delivers RAG-based search and can quickly surface information from FAQs in response to customer questions. However, embedding agentic AI into web and app experiences can deliver even better ROI because it takes action on behalf of customers, such as resetting their passwords or checking order status.”
Centralize access to customer data
Using AI in more interactive customer experiences requires using data that has been centralized and cleansed to train and validate AI agents. Companies use tools like customer data platforms and data fabrics to connect customer data and interactions.
“A robust AI-powered CX strategy is only as good as its underlying data and related governance, and any CX program should emphasize continuous testing and learning strategies to ensure data freshness and accuracy,” says Tara DeZao, senior director of product marketing and customer engagement at Pega. “This approach not only boosts agent performance but also mitigates risk and promotes brand equity as consumers interact with businesses across channels.”
When centralizing customer data, leaders must establish data controls around security, user access levels, and identity management. Many organizations utilize data security posture management (DSPM) platforms to reduce risks when they manage many data sources, multiple cloud database platforms, and disparate infrastructure.
“By rethinking how data is stored and accessed, moving from siloed third-party systems to user-centric data models, organizations can create more fluid, responsive web and mobile interactions that adapt to preferences in real-time,” says Osmar Olivo, VP of product management at Inrupt. “To maintain accuracy and performance, AI-driven experiences should be trained with diverse, real-world data while also incorporating user feedback mechanisms that allow individuals to correct, refine, and guide AI-generated insights by supplying their own preferences and metadata.”
Manish Rai, VP of product marketing at SnapLogic, predicts more than 80% of generative AI projects fail due to data connectivity, quality, and trust issues. “Success depends on tools that simplify agent development, make data AI-ready, and ensure reliability through observability, evaluation for accuracy, and policy enforcement.”
Rosaria Silipo, VP of data science evangelism at KNIME, notes many agentic applications have a human-in-the-loop step to check for correctness. “In other cases, special guardian AI agents focus on controlling the result; if the result is not satisfactory, they send it back and ask for an improved version.” For more data-related tasks, such as sentiment analysis, “genAI accuracy is compared to the accuracy of other classic machine learning models.”
Elevate customer service calls and chats to AI agents
Beyond information searches and fulfilling simple tasks are the customer service calls and chats that frustrate both consumers and the human agents serving them. In one survey, 23% of respondents claimed they would rather watch paint dry than go through repeated bad customer service experiences.
Instead of a rule-based chatbot with limited capabilities, customer service AI agents can sift through the data and respond to customers, while human agents tackle more challenging cases with the assistance of a customer success AI agent.
“There is a clear connection between customer satisfaction and the use of effective self-service,” says Vinod Muthukrishnan, VP and COO of Webex Customer Experience Solutions at Cisco. “The evolution towards truly agentic AI transforms self-service experiences by orchestrating end-to-end engagement between the brand and the customer. This advanced AI capability empowers customer experience teams to offer intelligent, seamless interactions, meeting customers where they are and on their schedules.”
Part of the challenge is data, and the other is that many customer experiences were developed as point solutions addressing only part of the customer journey. Technologists should apply design thinking approaches to re-engineer more holistic experiences when transforming to genAI-enabled engagements.
“Customer-facing applications such as websites, mobile apps, and B2C messaging typically have back-end integrations with customer-specific data sources that enable the application to answer questions and resolve problems,” says Chris Arnold, VP of customer experience strategy at ASAPP. “Leveraging an LLM to curate a personalized experience in a conversational format is far superior to the transactional experience offered by these applications alone.”
Fully test AI agents before deploying CX capabilities
Organizations looking to develop more advanced CX capabilities and autonomous AI agents will need a comprehensive testing plan to validate capabilities. Prompt filters, AI response moderation, content safeguards, and other guardrails help CX agents avoid conversations that are inappropriate or out of scope. However, brands must look beyond these basics and ensure that CX AI agents respond appropriately, accurately, and ethically.
“You can’t just throw an agent out there untested and unmonitored,” says Miles Ward, CTO of Sada. “Rigorous testing for accuracy and performance is non-negotiable. You need to know they’re delivering a frictionless, reliable experience, or you’re just creating a new set of problems.”
Ganesh Sankaralingam, data science and business analytics leader at LatentView, says AI experiences and LLM responses should be tested for accuracy and performance across five dimensions.
- Relevance: Measures how well the response is pertinent and related to the query.
- Groundedness: Assesses if the response aligns with the input data.
- Similarity: Quantifies how closely the AI-generated response matches the expected output.
- Coherence: Evaluates the flow of the response, ensuring it mimics human-like language.
- Fluency: Assesses the response’s language proficiency, ensuring it is grammatically correct and uses appropriate vocabulary.
Deon Nicholas of Forethought says, “Businesses should test AI experiences for accuracy and performance by running AI agents against historical customer questions and seeing how they do; measuring how often AI can handle customer interactions autonomously; and applying a separate evaluator model to check for conversation sentiment and accuracy.”
The future of AI agents in customer experience
How will AI agents impact CX in the near future? Mo Cherif, senior director of generative AI at Sitecore, recommends rethinking the experience entirely. “To create a genuinely agentic experience, don’t just enhance what already exists—build the journey specifically as a genAI-first interaction.”
There are contrary views on how AI agents will evolve. Some predict a more autonomous future where people empower and trust AI agents to make more complex decisions and take on a greater scope of actions. Others forecast a more human-centric approach, where AI agents augment human capabilities and partner with people to make smarter, faster, and safer decisions.
Michael Wallace, Americas solutions architecture leader for customer experience at Amazon Web Services, says agentic AI can resolve issues without human intervention. Consider a contact center that self-heals during a crisis, automatically redistributing resources, updating customer communications, and resolving issues before customers even experience them.
Wallace says, “Imagine an airline is facing a sudden traffic surge due to weather delays. With agentic AI, the contact center would make autonomous decisions about passenger rebooking and proactive notifications, allowing human agents to focus on complex customer needs rather than administrative tasks.”
Doug Gilbert, CIO and chief digital officer of Sutherland Global, says AI isn’t about automating customer experience; it should be about making experiences more human and intelligent. He says, “The true value of generative AI lies not in replacing human interactions but in enhancing them to be smarter, faster, and more natural. The secret is AI that learns from real-world interactions, constantly evolving to feel less robotic and more intuitive.”
Both autonomous and human-in-the-middle CX AI agents will likely materialize. In the meantime, businesses should fully research customer needs, improve data quality, and establish rigorous testing practices.
Java 25 to change Windows file operation behaviors 16 Jun 2025, 7:47 pm
As part of a quality outreach, some changes are coming in the planned Java 25 release with regard to file operations on Windows. The File.delete
command will no longer delete read-only files on Windows, and file operations on a path with a trailing space in a directory or file name will now fail consistently on Windows.
In a June 16 bulletin on Oracle’s inside.java blog, David Delabassee, Oracle director of Java relations, said File.delete
in JDK 25 has been changed on Windows so it now fails and returns false
for regular files when the DOS read-only attribute is set. Before JDK 25, File.delete
would delete read-only files by removing the DOS read-only attribute before deletion was attempted. But because removing the attribute and deleting the file are not a single atomic operation, this could result in the file remaining, with modified attributes. Applications that depend on the previous behavior should be updated to clear the file attributes before deleting files, Delabassee said.
To make the transition easier, a system property has been introduced to restore the previous behavior. Running File.delete
with -Djdk.io.File.allowDeleteReadOnlyFiles=true
will remove the DOS read-only attribute prior to deleting the file, restoring the legacy behavior.
Also in JDK 25, file operations on a path with a trailing space in a directory or file name now fail consistently on Windows. For example, File::mkdir
will return false
, or File::createNewFile
will throw IOException
, if an element in the path has a trailing space, because path names such as these are not legal on Windows. Prior to JDK 25, operations on a file created from such an illegal abstract path name could appear to succeed when they did not, Delabassee said.
Now in a rampdown phase, JDK 25, a long-term support (LTS) release, is due to be generally available September 16.
Databricks Data + AI Summit 2025: Five takeaways for data professionals, developers 16 Jun 2025, 8:19 am
At Databricks’ Data + AI Summit 2025 last week, the company showcased a variety of generative and agentic AI improvements it is adding to its cloud-based data lakehouse platform — much as its rival Snowflake did the previous week.
With the two competitors’ events so close together, there’s little time for their product engineering teams to react to one another’s announcements. But that also means they’re under pressure to announce products that are far from ready for market, so as to avoid being scooped, perhaps explaining the plethora of features still in beta-testing or “preview.”
Here are some of the key new products and features announced at the conference that developers and data professionals may, one day, get to try for themselves:
It’s automation all the way down
Many enterprises are turning to AI agents to automate some of their processes. Databricks’ answer to that is Agent Bricks, a tool for automating the process of building agents.
It’s an integral part of the company’s Data Intelligence platform, and Databricks s pitching it as a way to take the complexity out of the process of building agents, as most enterprises don’t have either the time or the talent to go through an iterative process of building and matching an agent to a use case. It’s an area that, analysts say, has been ignored by rival vendors.
Another way that Databricks is setting its agent-building tools apart from those of rival vendors is that it is managing the agent lifecycle differently — not from within the builder interface but via Unity Catalog and MLflow 3.0.
Currently in beta testing, the interface supports the Model Context Protocol (MCP) and is expected to support Google’s A2A protocol in the future.
Eliminating data engineering bottlenecks
Another area in which Databricks is looking to help enterprises eliminate the data engineering bottlenecks that slow down AI projects is in data management. It previewed a data management tool powered by a generative AI assistant, Lakeflow Designer, to empower data analysts to take on tasks that are typically processed by data engineers.
Lakeflow Designer could be described as the “Canva of ETL,” offering instant, visual, AI-assisted development of data pipelines, but Databricks also sees it as a way to make collaboration between analysts and engineers easier.
Integrated into Lakeflow Declarative Pipelines, it also supports Git and DevOps flows, providing lineage, access control, and auditability.
Democratizing analytics for business users while maintaining governance
Databricks also previewed a no-code version of its Data Intelligence platform called Databricks One that provides AI and BI tools to non-technical users through a conversational user interface.
As part of the One platform, which is currently in private preview and can be accessed by Data Intelligence platform subscribers for free, Databricks is offering AI/BI Dashboards, Genie, and Databricks Apps along with built-in governance and security features via Unity Catalog and the Databricks IAM platform.
The AI/BI Dashboards will enable non-technical enterprise users to create and access data visualizations and perform advanced analytics without writing code. Genie, a conversational assistant, will allow users to ask questions about their data using natural language.
Databricks has also introduced a free edition of its Data Intelligence platform — a strategy to ensure that more data professionals and developers are using the platform.
Integrating Neon PostgreSQL into the Data Intelligence platform
One month after acquiring Neon for $1 billion, the cloud lakehouse provider has integrated Neon’s PostgreSQL architecture into its Data Intelligence Platform in the form of Lakebase.
The addition of the managed PostgreSQL database to the Data Intelligence platform will allow developers to quickly build and deploy AI agents without having to concurrently scale compute and storage while preventing performance bottlenecks, simplifying infrastructure issues, and reducing costs.
Integrated AI-assisted data migration with BladeBridge
Databricks has finally integrated the capabilities of BladeBridge into the Data Intelligence platform, after acquiring the company in February, in the form of Lakebridge — a free, AI-assisted tool to aid data migration to Databricks SQL.
Earlier this month, Databricks rival Snowflake also introduced a similar tool, named SnowCovert, that uses agents to help enterprises move their data, data warehouses, business intelligence (BI) reports, and code to Snowflake’s platform.
Other updates from Databricks included expanded capabilities of Unity Catalog in managing Apache Iceberg tables.
The key to Oracle’s AI future 16 Jun 2025, 5:00 am
Oracle’s stock is finally catching up with its talk about cloud, fueled by surging infrastructure and AI demand. In its recent Q4 2025 earnings statement, the 46-year-old database giant surprised Wall Street with an 11% jump in revenue (to $15.9 billion) and bullish forecasts for the year ahead. Oracle’s stock promptly enjoyed its best week since 2001, leaping 24%. It’s relatively easy to please (and fool) investors, but there’s real substance in that 11% jump, and that substance is, at its foundation, all about data.
This is new for Oracle. I and others have criticized the company for being too slow to embrace cloud. As a result, both Microsoft Azure and Amazon Web Services pulled ahead of Oracle in database revenue a few years back as cloud became central to enterprise strategy.
Oracle, however, found a workaround.
For decades, Oracle Database has been the heart of the enterprise. It’s the system of record for much of the world’s most critical data. Instead of competing head-on with the likes of OpenAI and Google on foundation models, Oracle is offering something far more practical to its enterprise customers: the ability to run AI securely on their own data, within their own cloud environment. The new Oracle Database 23ai is a testament to this strategy, integrating AI capabilities directly into the database so that companies can get insights from their data without having to ship it off to a third-party service.
It’s smart, but it’s also not enough. Sustaining Oracle’s newfound momentum may require Oracle to succeed in an area where it has historically struggled: winning over developers. Developers, after all, are the people building the applications that will draw on all that Oracle data.
Cloud-based AI favors database vendors
Large language models (LLMs) have wowed us for the past few years with all they could do with massive quantities of publicly available data (even if the unwitting data providers, like Reddit, haven’t always been happy about that role). They’ve started to stumble, however, en route to true enterprise adoption, hampered by security concerns and the reality that as informed as they are by the internet, they’re incredibly dumb when it comes to a given enterprise because they have zero access to that data. Companies have tried to get around this problem with retrieval-augmented generation (RAG) and other tactics, but the real bonanza is waiting for the companies that can help enterprises apply AI to their data without having that data leave their secured infrastructure, whether running on premises or in the cloud.
A few vendors are figuring it out. Indeed, I’ve had a front-row seat to this phenomenon at MongoDB, where companies are finding it useful to store and search vector embeddings alongside operational data. Oracle is apparently discovering something similar, as noted on its earnings call, and is finally spending big on data centers to keep up with rising demand ($21 billion this year, which is more than 10 times what it was just four years ago).
Unsurprisingly, Oracle founder and CTO Larry Ellison couldn’t resist a bit of grandstanding on the earnings call, effectively arguing that when it comes to enterprise AI, Oracle has a home-field advantage. “These other companies say they have all the data, so they can do AI really well…. The only problem with that statement is they don’t have all the data. We do. We have most of the world’s valuable data. The vast majority of it is in an Oracle database.” It’s classic Ellison swagger, but there’s truth there: Oracle databases remain ubiquitous in large enterprises, storing troves of business-critical data.
Oracle is leveraging that position by making its database and cloud services “AI-centric.” Oracle’s pitch is that enterprises can use the data already sitting in their Oracle apps and databases to gain AI-driven insights securely and reliably without having to migrate everything to some new (and likely less secure) AI platform. “We are the key enabler for enterprises to use their own data and AI models,” Ellison argues. “No one else is doing that…. This is why our database business is going to grow dramatically.”
In Oracle’s view, the real gold rush in enterprise AI isn’t just hyping up the largest general AI models, but helping companies apply AI to their own data. Oracle has also been a bit less Oracle-like by embracing multicloud. Rather than insisting all workloads run on Oracle’s cloud (Oracle Cloud Infrastructure, or OCI), the company has inked partnerships to make Oracle Database available on AWS, Google Cloud, and Microsoft Azure. For enterprises concerned about data sovereignty, latency, or just avoiding single-vendor lock-in, that’s an attractive story.
But it’s not yet a complete story. For that, Oracle needs to figure out developers.
The missing piece
Eleven years ago I called out the missing piece in Oracle’s cloud ambitions: “Oracle … has built a cloud for its existing customers but not for developers who are building big data, internet of things, mobile, and other modern applications.” I doubled down on this theme, insisting that Oracle had failed to remedy “an almost complete lack of interest from developers.”
Plus ça change…
Oracle’s cloud growth so far has come largely from selling to the CIO, not the developer, signing big deals with companies that already trust Oracle for databases or apps, helping customers migrate existing Oracle-centric workloads, etc. That’s a perfectly valid strategy (and a lucrative one), but it leaves Oracle underexposed to the grassroots innovation happening in the broader developer community. Why does developer enthusiasm matter if Oracle can keep signing enterprise contracts? Because in the cloud era, developers are often the new enterprise kingmakers driving technology adoption.
Sure, the CIO of a large bank might sign a top-down deal with Oracle for an ERP system or database licenses, but it’s the developers and data scientists who are dreaming up the next generation of applications—the ones that don’t even exist yet. They have a world of cloud options at their fingertips. If they aren’t thinking of Oracle as a platform to create on, Oracle risks missing out on new workloads that aren’t already tied to its legacy footprint.
A modest proposal
It’s not my job to tell Oracle how to get its developer act together, but there are some obvious (if difficult) things it can and should do. Oracle doesn’t need to become “cool” in a Silicon Valley startup sense, but it does need to lower barriers so that a curious engineer or a small team can readily choose Oracle (including MySQL) for their next project. For example, years ago Oracle ceded MySQL’s dominant open source pole position to Postgres. It’s unclear whether the company would ever consider an open governance model for MySQL, but that may be the only way to put MySQL on equal footing. MySQL has great engineering, but it needs community.
Less culturally difficult, Oracle needs to keep improving onboarding and pricing to make it easy for developers to pick its cloud. One thing that made AWS popular with developers was the ability to swipe a credit card and get going immediately, with transparent usage-based pricing. Oracle has made progress here. OCI has a free tier and straightforward pricing for many services, but it can go further. For example, offering generous free credits for startups, individual developer accounts, or open-source projects could also seed grassroots adoption.
Oracle should also take a cue from Microsoft and increase its presence in developer communities and conferences, highlight success stories of “built on OCI” startups, and expand programs that engage developers directly. Oracle has started moving in this direction. At its CloudWorld 2024 conference, Oracle unveiled Oracle Code Assist (an AI-powered programming assistant for Java developers on OCI) and new features in its Oracle Kubernetes Engine to better support cloud-native apps and AI workloads. These are welcome additions. The key is to get such tools into developers’ hands and iterate based on feedback, which could be accomplished by an emboldened developer advocacy team.
Finally, Oracle should package its unique strengths in a way that allows developers to easily tap into them. For instance, Oracle’s expertise in databases and data management could be offered as developer-friendly services. The company’s new Oracle Database 23c has “AI” features (JSON relational duality, vector search, etc.) that sound great, but Oracle needs to ensure a developer can quickly provision a development instance of this database, load some data, and call an API or driver to start building an AI-powered app. In short, Oracle should make its cool tech easily accessible. If Oracle can give developers a taste of what its high-performance cloud and data systems can do—without a months-long enterprise procurement cycle—some will inevitably be impressed and advocate using Oracle for new projects.
Oracle is achieving something rare: a true second act in tech, decades into its life. By leaning into what it does best (databases, enterprise applications, and now high-performance cloud infrastructure) Oracle has made itself a contender in the age of AI. The next test will be whether the company can broaden its influence beyond the traditional enterprise IT shops that have always been its stronghold. If Oracle can find a way to engage developers on their own terms (without diluting its enterprise savvy), it could unlock another level of growth that makes today’s resurgence look modest.
Understanding how data fabric enhances data security and governance 16 Jun 2025, 5:00 am
Data fabric is a powerful architectural approach for integrating and managing data across diverse sources and platforms.
As enterprises navigate increasingly complex data environments, the need for seamless data access coupled with robust security has never been more critical. Data fabric has the potential to enhance both data security as well as governance, but it’s not always a straight path for organizations to achieve the optimal outcome.
Understanding data fabric architecture
To get the most of data fabric, it’s important to first actually understand what it is and what it can actually provide to an organization. Unfortunately, defining data fabric can also be a challenge.
[ Related: Data mesh vs. data fabric vs. data virtualization: There’s a difference ]
“Thanks to multiple, often vendor-centric, definitions, there remains confusion in the industry about the precise nature of data fabric,” Matt Aslett, director, with global technology research and advisory firm ISG, told InfoWorld.
ISG defines data fabric as a technology-driven approach to automating data management and data governance in a distributed architecture that includes on-premises, cloud and hybrid environments. Aslett added that a common misconception is that enterprises must discard existing data platforms and management products to embrace data fabric.
Key elements of a data fabric architecture include the following:
- Metadata-driven data identification and classification
- Knowledge graphs
- Automated, ML-driven, data management.
“These capabilities provide the connective tissue that traverses disparate data silos and can complement the use of existing bespoke data tools by facilitating an abstracted view of data from across the business to support business intelligence and artificial intelligence initiatives that rely on the data unification,” Aslett said.
Data security challenges
Implementing a data fabric architecture presents several security challenges that enterprisemust address to ensure the integrity, confidentiality and availability of data assets.
Among the security challenges are these six::
Data silos and fragmentation
Despite the promise of integration, many organizations struggle with persistent data silos in their initial data fabric implementations.
“The biggest challenge is fragmentation; most enterprises operate across multiple cloud environments, each with its own security model, making unified governance incredibly complex,” Dipankar Sengupta, CEO of Digital Engineering Services at Sutherland Global told InfoWorld.
Compliance and regulatory complexity
Adhering to industry standards and regulations such as General Data Protection Regulation (GDPR), Health Insurance Portability and Accountability Act (HIPAA) and California Consumer Privacy Act of 2018 (CCPA) is a significant challenge in data fabric implementations.
Different data types and sources may fall under different regulatory frameworks. As such, implementing consistent compliance measures across the entire fabric requires careful planning and execution.
Talent
According to Sengupta, the other blind spot is talent, with 82% of firms struggling to hire skilled data professionals.
Shadow IT
Shadow IT is also a persistent threat and challenge. According to Sengupta, some enterprises discover nearly 40% of their data exists outside governed environments. Proactively discovering and onboarding those data sources has become non-negotiable.
Data fragmentation
Another major obstacle to effective data security and governance is fragmentation.
“There are too many data silos to secure and govern, and too many tools required to get the job done,” Edward Calvesbert, Vice President, Product Management, for IBM watsonx.data, told InfoWorld.
IT complexity
According to Anil Inamdar, Global Head of Data Services at NetApp Instaclustr, the potential for data fabric security/governance challenges really begins with the complexity of the organization’s IT environment.
“If security is already inconsistent across hybrid or multi-cloud setups, teams will subsequently struggle to get their data fabric architecture as secure as it needs to be,” Inamdar said.
How data fabric enhances security
While there are some challenges, the reason why so many organizations choose to deploy data fabric is because it does significantly enhance data security and governance.
Data fabric architectures offer significant advantages for enhancing security when properly implemented across a number of different domains.
Centralized Security Policies
Organizations are using data fabric to fix the challenge of fragmentation. IBM’s Calvesbert noted that with data fabric organizations can create a centralized set of policies and rules capable of reaching all data within the organization. Policies and rules can be linked to any and all data assets through metadata like classifications, business terms, user groups, and roles – and then enforced automatically whenever data is accessed or moved.
Regulatory compliance
A data fabric deepens organizations’ understanding and control of their data and consumption patterns. “With this deeper understanding, organizations can easily detect sensitive data and workloads in potential violation of GDPR, CCPA, HIPAA and similar regulations,” Calvesbert commented. “With deeper control, organizations can then apply the necessary data governance and security measures in near real time to remain compliant.”
Metadata management
Automated metadata management and data cataloging are integral components and benefits of data fabric.
“It’s a big deal, because when metadata is automatically tagged and tracked across both cloud and on-prem environments, you are getting the level of visibility that is going to make security folks and compliance officers happy,” NetApp’s Inamdar commented. “Automating this process within a data fabric creates that digital breadcrumb trail that follows data wherever it goes.”
Automated data discovery and classification
Automated tools within data fabric discover and classify data, reducing manual effort and enhancing governance. This involves identifying sensitive data across environments, categorizing it and applying appropriate security measures.
Data access control and authorization
Data fabric supports granular access control through Role-Based Access Control (RBAC) and Attribute-Based Access Control (ABAC), ensuring only authorized users can access sensitive data. This is vital for minimizing unauthorized access risks, with mechanisms like dynamic masking complementing these controls.
Data encryption and masking
Data fabric facilitates data encryption for protection at rest and in transit and data masking to obscure sensitive information. Encryption transforms data so it’s not transparent and available for anyone to look at, while masking replaces data with realistic but fake values, ensuring privacy.
Data governance frameworks
Data fabric supports implementing and enforcing data governance frameworks, providing tools for policy definition, monitoring, and enforcement. This ensures data is managed according to organizational policies, enhancing control and accountability.
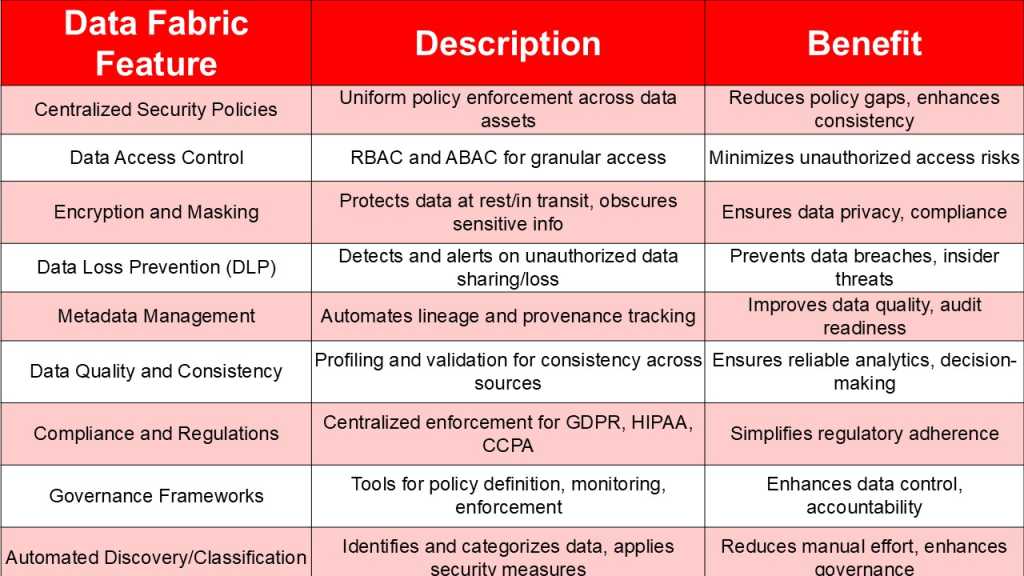
IDG
Why data validation is critical for data fabric success
Data security and governance inside a data fabric shouldn’t just be about controlling access to data, it should also come with some form of data validation.
The cliched saying “garbage-in, garbage-out” is all too true when it comes to data. After all, what’s the point of ensuring security and governance on data that isn’t valid in the first place?
“Validating the quality and consistency of data is essential to establishing trust and encouraging data usage for both BI and AI projects,” Alslett said.
So how can and should enterprises use data validation within a data fabric? Sutherland Global’s Sengupta commented that the most effective validation strategies he has seen start with pushing checks as close to the source as possible. He noted that validating data upfront —rather than downstream — has helped reduce error propagation by over 50% in large-scale implementations. This distributed approach improves accuracy and lightens the processing load later in the pipeline.
Machine learning is playing a growing role as well. Statistical baselines and anomaly detection models can flag issues that rigid rule-based systems often miss. In one case cited by Sengupta this approach helped increase trust in critical data assets by nearly 80%.
“What’s often overlooked, though, is the value of context-aware validation—cross-domain consistency checks can expose subtle misalignments that may look fine in isolation,” Sengupta said. “For real-time use cases, stream validation ensures time-sensitive data is assessed in-flight, with accuracy rates approaching 99.8%.”
Benefits and use cases: data fabric in the real world
The real world impact of data fabric is impressive. While it can often just be used as a marketing term by vendors, there is tangible return on investment opportunities too.
“In our work with large enterprises, the most tangible impact of data fabric initiatives comes from their ability to speed up access to trustworthy data, safely and at scale,” Sengupta said.
For instance, a global financial institution reduced its regulatory reporting time by 78% and accelerated access provisioning by 60% after re-architecting its data governance model around unified security policies.
In healthcare, a provider network improved patient data accuracy from 87% to 99%, while also cutting integration time for new data sources by 45%, a critical gain when onboarding new partners or navigating compliance audits.
A manufacturing client saw a 52% drop in supply chain data errors and significantly improved the processing of IoT sensor data, boosting integration speed by 68%.
In retail, better orchestration of policies and quality controls translated into 85% faster delivery of customer insights, a 3x increase in analyst productivity, and a 30% reduction in storage costs through better data hygiene.
“What these outcomes show is that when data is treated not just as an infrastructure component but as an enabler of business velocity, the returns are both measurable and strategic,” Sengupta said.
Top 6 multicloud management solutions 16 Jun 2025, 5:00 am
Multicloud is increasingly common in today’s enterprises. The use of multiple public clouds, even spreading applications across clouds, can help with compliance, boost availability, reduce risk, or avoid vendor lock-in. Companies also adopt multiple clouds to support best-of-breed strategies, or as the result of mergers or acquisitions.
Enterprises already use an average of 2.4 public cloud providers, according to Flexera’s 2025 State of the Cloud report. And multicloud adoption is anticipated to rise further, driven by new AI demands and the sovereign cloud movement. In addition to using multiple public clouds like Amazon Web Services (AWS), Microsoft Azure, or Google Cloud Platform (GCP), many enterprises today also rely on a mix of on-prem data centers, private clouds, bare-metal, and edge environments.
Multicloud management solutions help unify common functions across these environments, supporting deployment, configuration, governance, observability, monitoring, cost analysis, and more. No solution covers every area, though, which is why niche tools have emerged for deeper customization.
Below, we survey six top multicloud management systems for 2025. Some are cloud-provided, others cloud-agnostic. Some focus on infrastructure as code (IaC) or platform engineering, while others offer GUI-based control and enterprise bells and whistles. Some aim to provide all-purpose enterprise platforms, while others take a Kubernetes-first approach. Whatever your needs, the right fit is likely out there if you do some digging.
Google Cloud Anthos
Multicloud manager with hyperscaler muscle
Google Cloud Anthos is a full-featured managed platform that enables you to build and manage Kubernetes-based container applications in hybrid cloud and multicloud environments. Don’t let the name fool you—Anthos works with AWS, Azure, and GCP, as well as workloads on premises and at the edge.
Anthos provides a single control plane with declarative policies to ensure consistency across configuration management, service mesh, security, access policies, telemetry, and observability. You can group “fleets” to organize clusters and resources for multicloud administration.
At its core are open source projects like Kubernetes, Istio, Knative, and Tekton. Anthos can run anywhere and includes tooling to aid VM-to-container migration. It also provides a marketplace for third-party add-ons.
One hindrance—for fans of vanilla Kubernetes—could be its bias toward Google’s flavor of Kubernetes under the hood (Google Kubernetes Engine, or GKE). But that’s a small gripe, considering Google created Kubernetes and continues to lead its development.
If you’re looking for a strong option to deploy, observe, monitor, and govern workloads on-premises and across major clouds—with the same power as GCP—then Anthos should be on your short list.
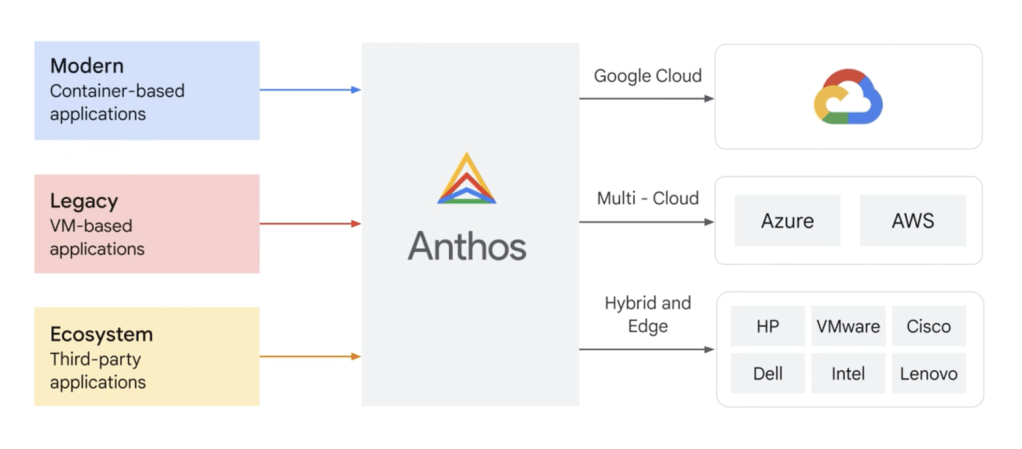
Google Cloud
HCP Terraform
Terraform with enterprise bells and whistles
Terraform is a command-line tool and configuration language for infrastructure as code (IaC). Inherently multicloud, Terraform automates all kinds of provisioning and management tasks, covering everything from cloud server, storage, network, and database resources to deployment pipelines, serverless applications, Kubernetes clusters, and much more.
Built on top of Terraform, HashiCorp’s HCP Terraform offers a hosted platform to standardize and collaborate on IaC at scale. It abstracts configuration management with features like registries for modules and secrets, policy enforcement, state storage, and governance controls. A self-hosted option is also available.
Terraform itself is cloud-agnostic and supports AWS, Microsoft Azure, Google Cloud, Oracle Cloud, Alibaba Cloud, and more. It’s mature and widely adopted, having helped power the devops and container movements since 2014.
Once open source, Terraform is now licensed under HashiCorp’s Business Source License (BSL), prompting the creation of OpenTofu as a community-driven alternative—and the departure of some core maintainers. Another challenge is that writing and maintaining Terraform modules at scale can be complex, even with a managed platform.
That said, Terraform is the de facto cross-cloud IaC standard. If you don’t mind some lock-in, HCP Terraform offers granular control over cloud resources with SaaS-like usability.
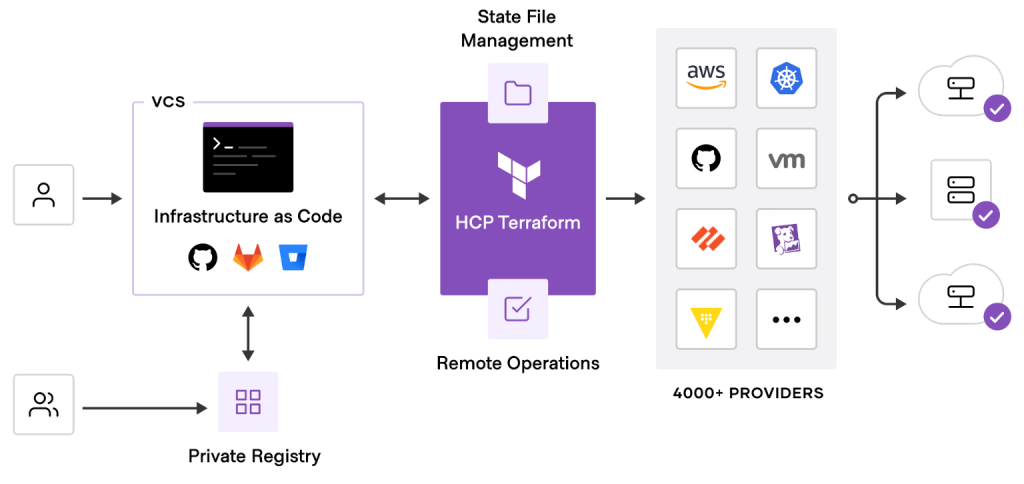
HashiCorp
HPE Morpheus Enterprise
Hybrid multicloud with wide enterprise coverage
HPE Morpheus Enterprise is a hybrid multicloud management solution that stands out for its broad feature coverage and support for both visual and programmable infrastructure automation.
Morpheus supports AWS, Microsoft Azure, Google Cloud Platform, IBM Cloud, Oracle Cloud, Nutanix, KVM, Kubernetes, and other niche clouds and surrounding technologies. It enables self-service provisioning, backups, compliance checks, and more—accessible via API, command-line interface, or GUI.
As you might expect from HPE, Morpheus is more tuned to the governance side of the multicloud equation than others on this list, with cost analytics, policy enforcement, and automation all part of the mix. Morpheus offers deep visibility and strong role-based access controls, making it a good choice for regulated environments.
HPE Morpheus Enterprise provides a flexible way to build various platform stacks, and integrates with a wide range of enterprise-oriented tools and services. However, Morpheus is a bit opinionated and not as built on open source as other options.
Morpheus makes sense for enterprises seeking a flexible, all-in-one solution for managing a hybrid infrastructure spanning on-prem data centers, edge deployments, and multiple clouds.

HPE
Humanitec
Multicloud deployment shifts to the developer
As platform engineering gains traction, internal developer platforms (IDPs) are reframing multicloud management, abstracting devops complexity and enabling self-service workflows. Humanitec leads this space, offering a cloud-agnostic infrastructure management layer.
Rather than prescribing tools, Humanitec serves as a “platform for platforms,” letting you stitch together your stack. Developers define workloads using Score, a workload spec, while the Platform Orchestrator generates configurations and populates a visual UI for deployment and management.
Humanitec is purpose-built for optimizing the cloud-native deployment-feedback loop, and it can be configured to work across all major clouds. But, it isn’t focused on cost visibility or legacy VM orchestration like traditional multicloud suites, and it doesn’t replace observability or security tools.
If your platform team wants to build a flexible, developer-friendly layer for cross-cloud deployments and operations, Humanitec is a strong choice. That said, it’s not alone—alternatives include Port, Crossplane, and Backstage, each offering different flavors of the IDP approach.
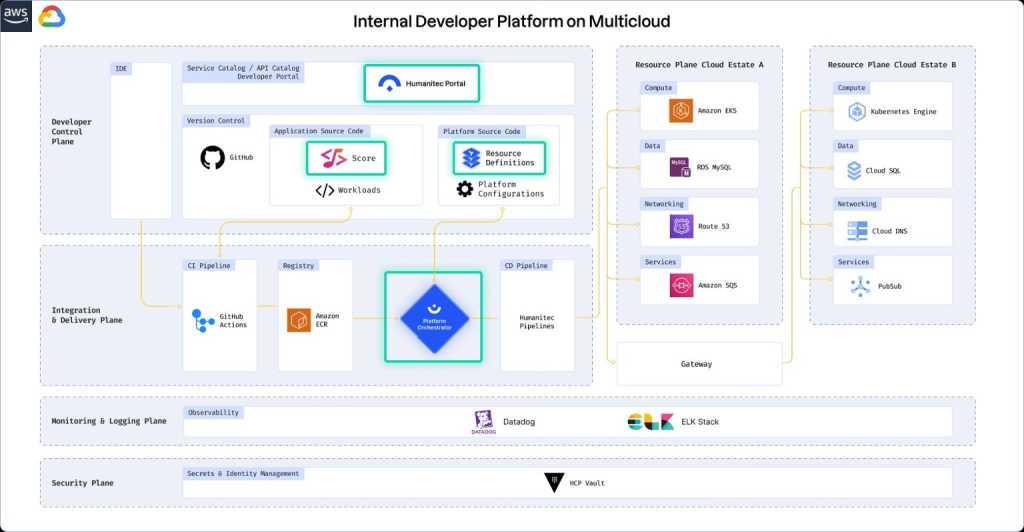
Humanitec
Nutanix Cloud Platform
A single pane of glass for hybrid multicloud
Nutanix is a hybrid multicloud platform that is quite universal—it can be used to run, manage, and secure virtual machines and containers across public clouds, private data centers, and edge environments.
Nutanix Cloud Platform consolidates functionality typically spread across multiple tools into a single control plane. It offers cost analysis, Kubernetes management, data security, monitoring, self-healing, disaster recovery, and more.
Nutanix Cloud Platform is highly tuned for deploying and migrating enterprise apps and databases across environments. You choose your hardware, hypervisor, cloud provider, and Kubernetes platform, and the Nutanix platform stitches it all together. It also includes automation to optimize performance and detect security anomalies across clouds.
Nutanix Cloud Platform is GUI-first and opinionated, emphasizing “click ops” over code. This can be a plus for IT teams without established IaC workflows, but may turn off devops engineers looking for code-based, declarative control.
If you need broad feature coverage in a platform that supports on-prem and multicloud, and you prefer a managed experience over heavy scripting, Nutanix is a strong choice.
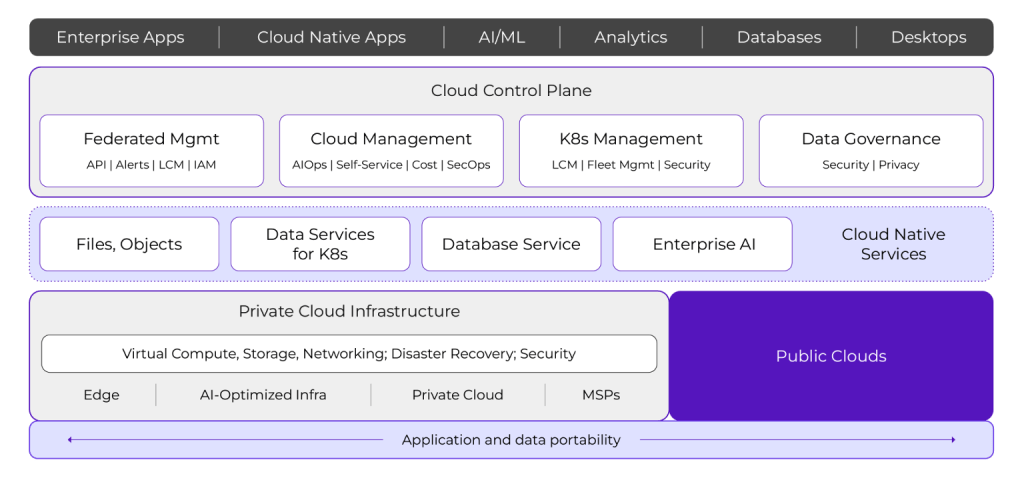
Nutanix
Palette
Kubernetes-first malleable multicloud operations
It didn’t take long for Kubernetes to become the cloud-agnostic container engine of choice in the enterprise. Palette, by Spectro Cloud, is a strong option for enterprises building on Kubernetes that require a multicloud management layer.
Palette provides a modular architecture for managing cluster operations across the stack, including deployment, security policies, networking, and monitoring. It supports all major clouds as well as bare metal, edge, and data center deployments, using a declarative model based on the Cloud Native Computing Foundation’s Cluster API (CAPI).
Spectro Cloud provides “packs,” a layered stack concept that supports a wide range of integrations for areas like authentication, ingress, edge Kubernetes, networking, security, service mesh, and more. This enables best-of-breed customization for specific needs.
While Spectro Cloud enforces configurations, policies, and life cycle constraints, deep governance and cost analysis are out of scope.
Palette is a great option for devops-heavy, Kubernetes-native organizations that span multiple clouds and need a malleable solution to unify operations and delivery across environments.
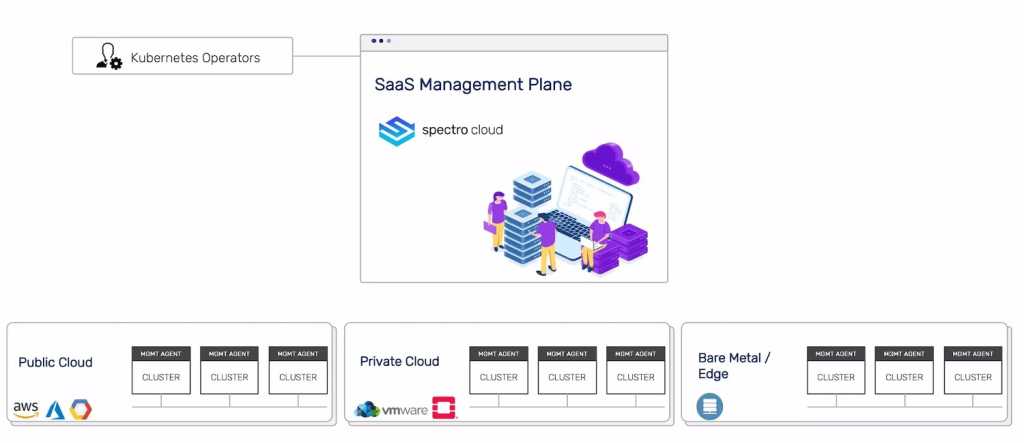
Spectro Cloud
Honorable mentions
There are a number of other platforms that get the broad multicloud sticker, because they work across clouds and have 80% of the expected features. SUSE Rancher and Cloudify are two other mature, all-in-one examples with proven industry use. Early-stage players aiming to orchestrate multicloud operations include Firefly, Fractal Cloud, and emma.
Plenty of alternative Kubernetes platforms (like Platform9, Mirantis, and D2iQ) support public and private cloud deployments. For VMware-heavy organizations, VMware Cloud Foundation (VCF) from Broadcom offers a platform to manage VMware-based workloads across private and VMware-aligned public cloud environments.
And there are plenty of niche tools to fill the gaps. As we’ve seen, many multicloud management platforms focus on deployment and configuration, leaving out functions like cost analysis and policy enforcement. These specialized tools cover those gaps and others:
- Cost analysis and optimization: Flexera Cloud Management Platform, Cloudbolt, CloudHealth by VMware, CloudZero, Apptio Cloudability, OpenCost, and Kion
- Deployment and GitOps: ArgoCD, Spacelift, Harness, Red Hat Ansible, and Scalr
- Policy, governance, and security: Open Policy Agent (OPA), Fugue, Prisma Cloud, and Lacework
- Monitoring and observability: Datadog, New Relic, Grafana Cloud, and Dynatrace
A moving target
73% of organizations are using two or more clouds, according to a 2025 survey of 500 IT leaders by HostingAdvice.com. Yet, while multicloud is now commonplace, true multicloud management is more elusive. Each cloud has its own nuances, making a truly agnostic, fully-featured control plane for deploying and managing workloads a dream we’re still chasing.
Because the industry keeps swinging between monolithic “all-in-one” platforms and best-of-breed microservices for niche tasks, it’s hard to decipher where cloud management approaches will ultimately land. It’s also increasingly iffy to recommend open-source options as a sure thing, given ongoing license changes and ecosystem fragmentation.
Thankfully, the platforms discussed above can take you a good way toward multicloud usage, abstracting much of the complexity and offering unified control plane options for your estates. Of course, this comes with tradeoffs—namely, vendor lock-in and giving up some control over building your own platform. That’s one reason platform engineering has emerged as a key practice.
Looking ahead, cloud-agnostic layers to manage these experiences will remain essential—and they’ll continue to evolve with each new technology wave.
Rethinking the dividing lines between containers and VMs 16 Jun 2025, 5:00 am
The technology industry loves to redraw boundary lines with new abstractions, then proclaim that prior approaches are obsolete. It happens in every major arena: application architectures (monoliths vs. microservices), programming languages (JVM languages vs. Swift, Rust, Go), cloud infrastructure (public cloud vs. on-prem), you name it.
False dichotomies are good at getting people excited and drawing attention, and they make for interesting debates on Reddit. But nearly without exception, what tends to happen in tech is a long period of co-existence between the new and the old. Then usually the old gets presented as new again. Monolithic application architectures sometimes prevail despite modern theology around microservices. On-prem data centers have not, in fact, been extinguished by public clouds. Serverless has not killed devops. The list goes on.
I think the most interesting false dichotomy today is the supposed line between virtual machines (VMs) and containers. The former has been maligned (sometimes fairly, sometimes not) as expensive, bloated, and controlled by a single vendor, while the latter is generally proclaimed to be the de facto application format for cloud-native deployments.
But the reality is the two worlds are coming closer together by the day.
Now more than 10 years into the rise of containers, the relationship between containers and VMs can be better described in terms of melding, rather than replacement. It’s one of the more nuanced evolutionary themes in enterprise architecture, touching infrastructure, applications, and, most of all, security.
The rise of containers
The lineage of containers and virtual machines is rather involved. Linux namespaces, the primitive kernel components that make up containers, began in 2006. The Linux Containers project (LXC) dates back to 2008. Linux-vserver, an operating system virtualization project similar to containers, began in 1999. Virtuozzo, another container tech for Linux that uses a custom Linux kernel, was released as a commercial product in 2000 and was open-sourced as OpenVZ in 2005. So, containers actually predate the rise of virtualization in the 2000’s.
But for most of the market, containers officially hit the radar in 2013 with the introduction of Docker, and started mainstreaming with Docker 1.0 in 2015. The widespread adoption of Docker in the 2010’s was a revolution for developers and set the stage for what’s now called cloud-native development. Docker’s hermetic application environment solved the longstanding industry meme of “it works on my machine” and replaced heavy and mutable development tools like Vagrant with the immutable patterns of Dockerfiles and container images. This shift enabled a new renaissance in application development, deployment, and continuous integration (CI) systems. Of course, it also ushered in the era of cloud-native application architecture, which has experienced mass adoption and has become the default cloud architecture.
The container format was the right tech at the right time—bringing so much agility to developers. Virtual machines by comparison looked expensive, heavyweight, cumbersome to work with, and—most damning—were thought of something you had to wait on “IT” to provision, at a time when the public clouds made it possible for developers to simply grab their own infrastructure without going through a centralized IT model.
The virtues of virtual machines
When containers were first introduced to the masses, most virtual machines were packaged up as appliances. The consumption model was generally heavyweight VMware stacks, requiring dedicated VM hosts. Licensing on that model was (and still is) very expensive. Today, when most people hear the term “virtualization,” they automatically think of heavyweight stacks with startup latency, non-portability, and resource inefficiency. If you think of a container as a small laptop, a virtual machine is like a 1,000 pound server.
However, virtual machines have some very nice properties. Over time there’s been interest in micro-VMs, and a general trend toward VMs getting smaller and more efficient. The technology in the Linux kernel has evolved to the point where you can reasonably run a customer application in a separate kernel. These evolutionary gains have made VMs much more friendly as a platform, and today virtual machines are all around us.
On Windows, for example, if you install on normal hardware, you are running on virtual machines for security purposes. It has become commonly accepted that hypervisors are a powerful way to do security. Containers running in virtual machines can run on any cloud environment, and no longer just the private environment of the hypervisor providers.
Containers and VMs join forces
The need for multi-tenancy and the lack of a good security isolation boundary for containers are the primary reasons why containers and virtual machines are coming together today.
Containers don’t actually contain. If you’re running multiple workloads and apps inside a Kubernetes cluster, they’re all sharing the same OS kernel. So should a compromise happen to one of those containers, it could be a bad day for every other container plus the infrastructure that runs them.
Containers by default provide an OS-level virtualization that provides a different view of the file system and processes, but if there are any exploits in the Linux kernel, you can then pivot to the entire system, get privilege escalation, and either escape the container or execute processes in a process or context you shouldn’t be allowed to.
So, one of the fundamental reasons why the container and virtual machine worlds are colliding is that virtualized containers (aka, “virtual machine containers”) allow each container to run in its own kernel, with separate address spaces that do not touch the same Linux kernel the host system uses.
While security isolation in multi-tenant environments is the “hair on fire” reason why virtual machine containers are on the rise, there are also broad economic reasons challenging the boundaries between virtual machines and containers. When you have a virtual machine, you can assign specific memory requirements or specific CPU limitations in a more granular way and even apply usage policies at a global level.
Introducing Krata
The container format is a nearly 20-year-old evolution in how modern applications are created and operated across distributed cloud infrastructure. Although virtual machines were supposed to wither as the world adopted containers, the reality is that VMs are here to stay. Not only do virtual machines still have a large installed base, but developers are drawing on VM design principles to address some of containers’ still-turbulent challenges.
Krata, an open-source project by Edera, pairs a Type 1 hypervisor (the Xen microkernel) with a control plane that has been reimagined and rewritten in Rust to be container native. Krata provides the strong isolation and granular resource controls of virtual machines while supporting the developer ergonomics of Docker. It also creates an OCI-compatible container runtime on Kubernetes. This means Krata doesn’t require KubeVirt for virtualization; the system firmware boots and hands off to the Xen microkernel, which sets up interrupts and memory management for virtual machines that run pods in Kubernetes.
Because Krata uses a microkernel, it doesn’t make any changes to your existing Kubernetes operating system. You can use any OS—AL2023, Ubuntu, Talos Linux, etc.—and you don’t need KubeVirt to handle virtualization. Your containers run side-by-side on Kubernetes with no shared kernel state, preventing data access between workloads even in the event of kernel vulnerabilities.
Containers give developers flexibility, speed, and simpler deployment. Virtual machines offer superior workload isolation and security. For years, developers have been forced to choose. That’s changing.
Alex Zenla is co-founder and CTO of Edera.
—
New Tech Forum provides a venue for technology leaders—including vendors and other outside contributors—to explore and discuss emerging enterprise technology in unprecedented depth and breadth. The selection is subjective, based on our pick of the technologies we believe to be important and of greatest interest to InfoWorld readers. InfoWorld does not accept marketing collateral for publication and reserves the right to edit all contributed content. Send all inquiries to doug_dineley@foundryco.com.
SmartBear unveils AI-driven test automation for iOS and Android apps 13 Jun 2025, 5:49 pm
SmartBear has launched Reflect Mobile, an AI-powered, no-code, test automation tool kit that enables QA professionals to test native apps across the iOS and Android mobile platforms.
Announced June 11, Reflect Mobile features SmartBear’s HaloAI AI technology and extends SmartBear’s Reflect test automation solution, which was originally focused on web apps, to native mobile apps, SmartBear said. Reflect Mobile uses generative AI and record-and-replay to make test creation intuitive, fast, and codeless, according to the company. Reflect Mobile supports frameworks such as Flutter and React Native, enabling cross-platform testing via a single solution.
With Reflect Mobile, SmartBear advances its SmartBear Test Hub strategy, which brings API, web, and mobile testing into a unified solution, and helps teams simplify the testing of apps. Non-technical testers can build and maintain mobile test automation without needing scripting skills or engineering support. Built-in integrations for test management, device grid providers, and CI/CD pipelines enable Reflect Mobile to fit into existing QA and development environments, SmartBear said.
With the introduction of Reflect Mobile, SmartBear is marking a strategic expansion into the growing mobile-first market. SmartBear acquired Reflect in early-2024. Since then, SmartBear has integrated Reflect natural language test creation, visible insight capabilities, and AI automation into its suite of test automation solutions.
Visual Studio Code bolsters MCP support 13 Jun 2025, 4:58 pm
Visual Studio Code 1.101, aka the May 2025 version of Microsoft’s popular code editor, expands support for the Model Context Protocol, a technology that standardizes how applications provide context to large language models.
VS Code 1.101 was released June 12. Downloads are available for Windows, Mac, and Linux.
With the new release, VS Code has expanded its support for MCP-enabled agent coding capabilities with support for prompts, resources, sampling, and authenticating with MCP servers. Prompts, which can be defined by MCP servers to generate reusable snippets or tasks for the language model, are accessible as slash commands in chat. Developers can enter plain text or include command output in prompt variables.
With VS Code’s MCP resource support, which includes support for resource templates, resources returned from MCP tool calls become available to the model, can be saved in chat, and can be attached as context via the Add Context button in chat. Developers can browse resources across MCP servers, or for a specific server, by using the MCP: Browse Resources command, or using the MCP: List Servers command.
VS Code 1.101 offers experimental support for sampling, which allows MCP servers to make requests back to the model, and now supports MCP servers that require authentication, enabling interaction with an MCP server that operates on behalf of a user account for that service. Developers now can enable development mode to debug MCP servers by adding a dev
key to the server config.
Finally, new MCP extension APIs enable extensions to publish collections of MCP servers. This means VS Code extension authors can bundle MCP servers with their extensions or build extensions that dynamically discover MCP servers from other sources.
Other new features and improvements in VS Code 1.101:
- Developers now can define tool sets to use in chat, either through a proposed API or through the UI. Tool sets make it easier to group related tools together, and quickly enable or disable them in agent mode, Microsoft said.
- Selecting a history item in the Source Control Graph now reveals the resource for that item. Developers can choose between a tree view or list view representation from the
...
menu. - Language server completions now are available in the terminal for interactive Python REPL sessions.
- Task
runoptions
now has anInstancePolicy
property that determines what happens when a task reaches itsInstancelimit
.
GitHub launches Remote MCP server in public preview to power AI-driven developer workflows 13 Jun 2025, 9:48 am
GitHub has unveiled its Remote MCP server in public preview, allowing developers to integrate AI assistants like GitHub Copilot into their workflows without setting up local servers.
With just a click in Visual Studio Code or a URL in MCP-compatible hosts, coders can access live GitHub data — including issues, pull requests, and code — in real time, GitHub said in a blog post.
Unlike its local counterpart, the remote server is hosted by GitHub but retains the same codebase. It supports secure OAuth 2.0 authentication with Personal Access Tokens as a fallback, making adoption easier while maintaining control.
“This shift gives enterprises backend flexibility while preserving the familiar Codespaces UI,” said Nikhilesh Naik, associate director at QKS Group. “It decouples the orchestration control plane from GitHub’s infrastructure, a key step for hybrid developer setups.”
The technology builds on the Model Context Protocol, or MCP, which Keith Guttridge, VP Analyst at Gartner, described as “an emerging but crucial standard for AI tool interoperability.” While currently focused on developer experience, Guttridge noted MCP is evolving rapidly, with enterprise-grade features such as registry support and enhanced governance on the roadmap.
MCP’s growth mirrors broader industry demands, said Dhiraj Pramod Badgujar, senior research manager at IDC Asia/Pacific. “Self-hosted remote execution aligns with hybrid DevOps trends, balancing speed with security, especially for compliance-driven workflows.”
Hybrid workflows and enterprise flexibility
The Remote MCP server is designed to meet enterprise needs for hybrid development environments, particularly in sectors with strict compliance or network rules.
“GitHub’s shift to externalize the Remote MCP server is a technically deliberate response to enterprise demands for hybrid developer infrastructure,” Naik said, “where development workflows must align with internal network boundaries, compliance constraints, and infrastructure policies.”
The server allows enterprises to run development containers on self-managed Kubernetes clusters or virtual machines, integrating seamlessly with internal DNS, secret management, and CI/CD pipelines, positioning GitHub as a modular layer for internal developer platforms.
For industries handling sensitive intellectual property, the server aligns with GitHub’s existing policy framework. Guttridge said it “simplifies the process of enabling AI tools to interact with GitHub Services” without changing protections for regulated sectors.
Badgujar noted that enterprises prioritize internal control to strengthen security and manage cloud costs. “This supports DevSecOps by standardizing environments and aligning DevSecOps with governance policies,” he said.
The server currently requires the Editor Preview Policy for Copilot in VS Code and Visual Studio. It does not support JetBrains, Xcode, and Eclipse IDEs, which still rely on local MCP servers.
GitHub’s ecosystem advantage
Compared to competitors, GitHub’s remote MCP server leverages its ecosystem strengths. Naik argued that it shares GitHub Coder’s self-hosting capabilities but integrates more tightly with GitHub’s repositories and Codespaces containers for consistent environments, while Gitpod offers greater platform-agnostic flexibility.
Badgujar said GitHub balances execution and uniformity across Actions and Codespaces, unlike GitLab’s full-stack DevSecOps approach or Atlassian’s cloud-first focus.
Toward modular, secure development
Self-hosting the Remote MCP server reduces exposure to external systems but increases internal responsibilities.
“Though running in-house developer environments reduces external risk,” Naik said. “ But it pushes a lot of responsibility onto internal teams.”
Misconfigured permissions or weak container isolation could expose vulnerabilities, requiring robust sandboxing, audit trails, and secure integration with tools like artifact repositories and secret stores.
Guttridge cautioned that MCP, while promising, is still maturing. “It needs to evolve towards a more enterprise-ready standard with regard to registry, discovery, and governance,” he said, urging careful adoption in trusted environments.
Still, the architecture paves the way for modular, cloud-agnostic platforms. By decoupling the Codespaces frontend from backend orchestration, it enables workspaces to run across cloud VMs, bare-metal clusters, or edge nodes.
“This fosters API-driven, stateless environments with components like language servers and debuggers,” Naik said. Badgujar echoed that view, adding: “MCP has the potential to become the de facto standard of connecting AI-enabled systems together as it matures, helping reduce vendor lock-in.”
The GitHub MCP server repository is now available for developers to test during the preview period. While early in development, the Remote MCP Server marks a significant step toward modular, AI-powered developer platforms.
New Python projects to watch and try 13 Jun 2025, 5:00 am
Get a first look at the new Python Installation Manager for Windows, or try your hand at developing AI agents with Google’s Agent Development Kit for Python, or check out template strings in Python 3.14. Would you rather debate virtual threads in Python, or catch up on the May 2025 Python Language Summit? We’ve got that, too. It’s all here (and more) in this week’s Python Report.
Top picks for Python readers on InfoWorld
Get started with the new Python Installation Manager
At last, we have a native Python tool for installing, removing, and upgrading editions of the language in Microsoft Windows! It’s still in beta, but Python Installation Manager is set to replace py
before long. Why not give it a spin now?
How to deploy AI agents with the Google Agent Development Kit for Python
Google’s newly released toolkit for Python (and Java) eases you into writing complex, multi-step AI agents. And it doesn’t matter whether you’re using Google’s AI or someone else’s.
Python 3.14’s new template string feature
Once upon a time, there were f-strings for formatting variables in Python, and they were good … mostly. Now Python 3.14 introduces t-strings, or “template strings,” for a range of variable-formatting superpowers that f-strings can’t match.
How to use Marimo, a better Jupyter-like notebook system for Python
Jupyter Notebooks may be a familiar and powerful tool for data science, but its shortcomings can be irksome. Marimo offers a Jupyter-like experience, but it’s more convenient, interactive, and deployable.
More good reads and Python updates elsewhere
NumPy 2.3 adds OpenMP support
Everyone’s favorite Python matrix math library now supports OpenMP parallelization, although you’ll have to compile NumPy with the -Denable_openmp=true
flag to use it. This is the first of many more future changes to NumPy adding support for free-threaded Python.
Pyfuze: Package Python apps into single executables
This clever project uses uv
to conveniently deliver Python apps with all their dependencies included in a single redistributable package. You can even download and install the needed bits at setup time for a smaller initial package.
Does Python need virtual threads?
Python’s core developers are deciding now whether to add this Java-esque threading feature to Python. Do we need yet another concurrency feature on top of regular threads, async, multiprocessing, and subinterpreters in Python? You decide.
The Python Language Summit 2025
When Python’s best and brightest gathered in Pittsburgh this past May, they spoke of many things. This collection of blog posts offers a recap of topics such as fearless concurrency, the state of free-threaded Python, Python on mobile devices, and more.
Page processed in 0.948 seconds.
Powered by SimplePie 1.3.1, Build 20121030175403. Run the SimplePie Compatibility Test. SimplePie is © 2004–2025, Ryan Parman and Geoffrey Sneddon, and licensed under the BSD License.